Supervised fine-tuning (SFT)
by Stephen M. Walker II, Co-Founder / CEO
What is supervised fine-tuning?
Supervised fine-tuning (SFT) is a technique used to adapt a pre-trained (base) Large Language Model (LLM) to a specific downstream task using labeled data. The majority of LLMs used in 2024 are fine-tuned for chat or instruction-based interactions.
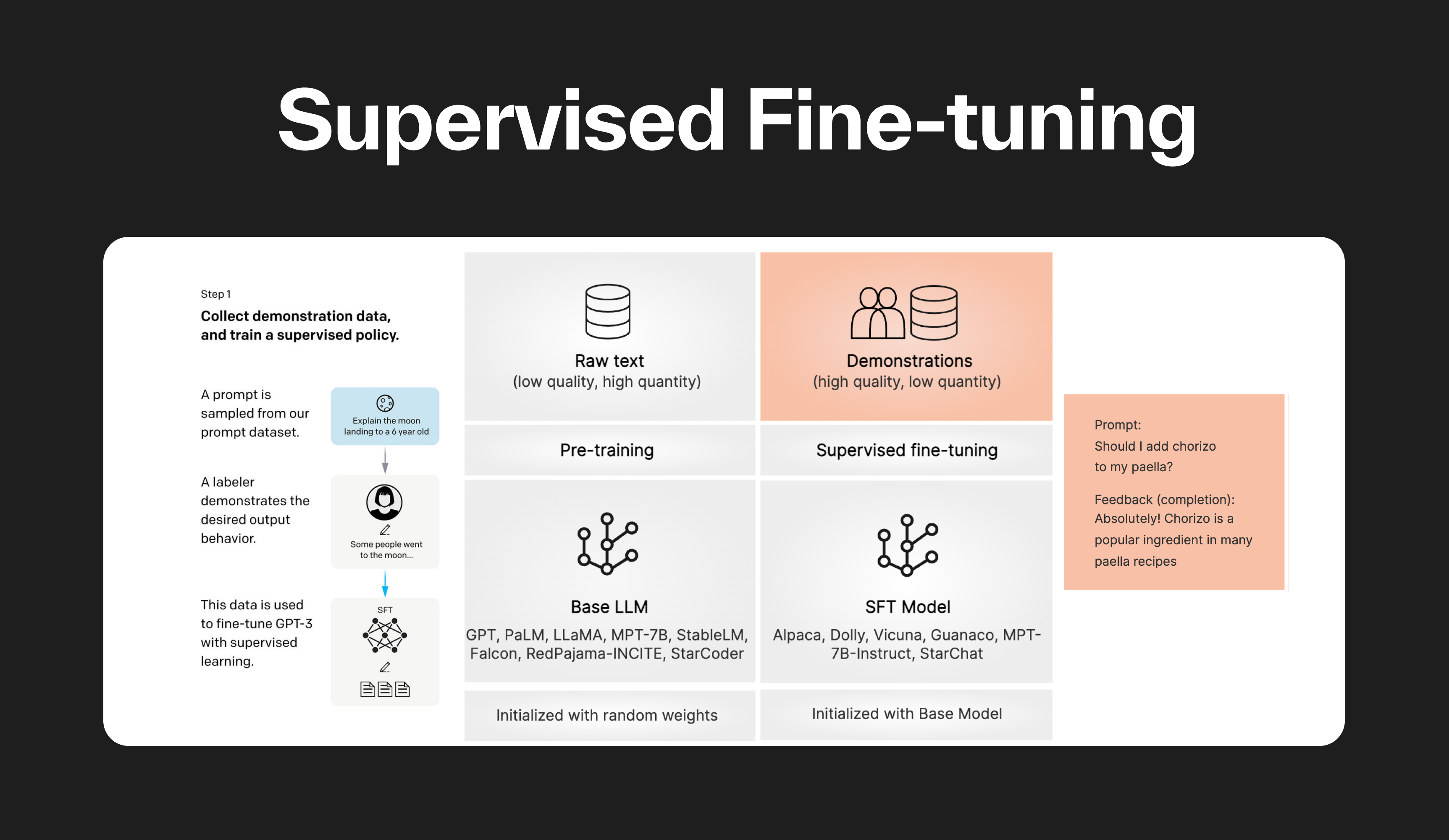
In SFT, the pre-trained LLM is fine-tuned on a labeled dataset using supervised learning techniques. The model's weights are adjusted based on the gradients derived from the task-specific loss, which measures the difference between the LLM's predictions and the ground truth labels.
This process allows the model to learn task-specific patterns and nuances by adapting its parameters according to the specific data distribution and task requirements.
SFT is typically done after model pre-training and is used to teach the model how to follow user-specified instructions. It is more computationally expensive than unsupervised fine-tuning but is also more likely to achieve better performance.
The amount of fine-tuning required depends on the complexity of the task and the size of the dataset. For simple style transfer using OpenAI models such as GPT-3.5 or GPT-4, typically 30-50 high-quality examples can yield excellent results.
To transform a base Large Language Model (LLM) into an instruction-following LLM (e.g., from Mistral to Mistral Instruct), it typically requires training with tens of thousands of examples.
Zephyr 7b fine-tune training was conducted on 16 Nvidia A100 GPUs for approximately 4 hours, which serves as an example starting point for models with 7 billion parameters.
How does supervised fine-tuning work?
Supervised fine-tuning is a three-step process used in AI and Large Language Models (LLMs) to optimize models for specific tasks.
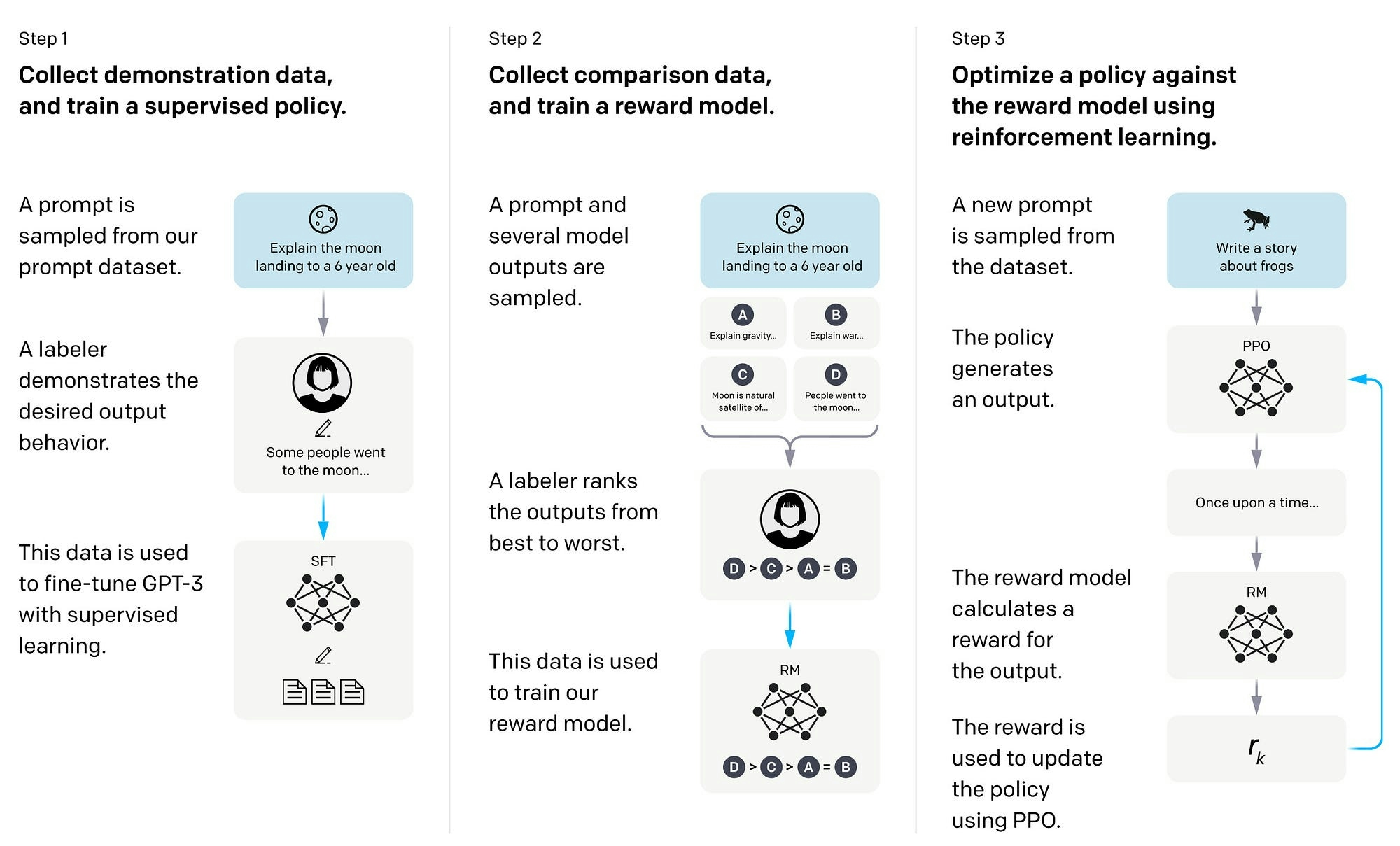
-
Step 1: Pre-training — The base or foundation model is initially trained on a large dataset, learning to understand language patterns, grammar, and context by predicting the next word in a given sentence. This stage helps the model to develop a wide-ranging understanding of language.
-
Step 2: Data Labeling — The dataset used for fine-tuning is prepared. Each data point is labeled with the correct output or answer. This labeled data is crucial for supervised learning, as it guides the model in adjusting its parameters during the fine-tuning process.
-
Step 3: Fine-tuning — The pre-trained model is then further trained on a task-specific dataset with labelled data. The model adjusts its parameters to improve its performance on this specific task. These tasks could range from text classification, sentiment analysis, to question-answering systems.
The term "supervised" is used because this process leverages labelled data, where the correct outputs are already known, to guide the learning process. This method enhances the model's accuracy by applying the broad language understanding from pre-training to a focused, specific task during fine-tuning.
Tuning Method | Description | When to Use |
---|---|---|
Supervised Fine-Tuning | Trains on labeled data. | Use when you have labeled data and need to adapt a model to a specific task for improved accuracy. |
Unsupervised Tuning | Trains without labeled data. | Use when labeled data is unavailable, and you want the model to learn from the data's inherent structure. |
Self-Supervised Tuning | Model generates labels from input data. | Use when you want to leverage large amounts of unlabeled data to pre-train models for downstream tasks. |
Reinforcement Learning | Trains with reward-based decisions. | Use when the task involves sequential decision-making and feedback is available to guide learning. |
What are the benefits of supervised fine-tuning?
Supervised fine-tuning (SFT) is a powerful technique used to adapt pre-trained Large Language Models (LLMs) to specific downstream tasks using labeled data. The benefits of supervised fine-tuning include:
-
Task-specific patterns and nuances — SFT allows the model to learn task-specific patterns and nuances by adapting its parameters according to the specific data distribution and task requirements.
-
Improved performance — Fine-tuning a pre-trained model enables it to leverage the knowledge and representations learned from vast amounts of data, leading to improved performance on specific tasks.
-
Data efficiency — SFT can be applied to a wide range of real-world scenarios, even when the number of labeled examples is limited, making it more data-efficient.
-
Resource efficiency — Fine-tuning pre-trained models saves substantial time and computational resources that would otherwise be required to train a model from scratch.
-
Customization — SFT allows for the adaptation of an LLM's behavior, writing style, or domain-specific knowledge to specific nuances, tones, or terminologies, offering deep alignment with particular styles or expertise areas.
-
Reduced overfitting — Techniques like early stopping, dropout, and data augmentation can be employed during fine-tuning to mitigate the risk of overfitting on small datasets and promote generalization to new data.
What are some common supervised fine-tuning techniques?
Common supervised fine-tuning methods for Large Language Models (LLMs) include LoRA (Low-Rank Adaptation) and its memory-efficient variant, QLoRA (Quantized LoRA). These techniques are part of the Parameter-Efficient Fine-Tuning (PEFT) family, which aims to make fine-tuning more efficient and accessible.
LoRA's approach to fine-tuning uses low-rank decomposition to represent weight updates with two smaller matrices, reducing the number of trainable parameters and making fine-tuning more efficient. This method has been shown to outperform full fine-tuning in some cases while avoiding catastrophic forgetting, a phenomenon that occurs when the knowledge gained during pre-training is lost during fine-tuning.
- LoRA (Low-Rank Adaptation) — A parameter-efficient fine-tuning technique that uses low-rank decomposition to represent weight updates with two smaller matrices, reducing the number of trainable parameters.
- QLoRA (Quantized LoRA) — A memory-efficient variant of LoRA that further reduces the memory requirements for fine-tuning large LLMs.
Additional supervised fine-tuning techniques for Large Language Models (LLMs) include:
-
Basic hyperparameter tuning — This involves manually adjusting the model's hyperparameters, such as the learning rate, batch size, and the number of epochs, until the desired performance is achieved.
-
Transfer learning — This technique involves fine-tuning a pre-trained LLM on a specific downstream task using labeled data.
-
Multi-task learning — This approach trains the LLM on multiple tasks simultaneously, allowing the model to learn task-specific patterns and nuances.
-
Few-shot learning — This technique involves training the LLM on a small number of labeled examples for a specific task, leveraging the model's pre-existing knowledge to make predictions.
-
Task-specific fine-tuning — This method involves training the entire model on task-specific data, allowing all the model layers to be adjusted during the training process.
-
Reward modeling — This technique involves training the LLM on a task-specific labeled dataset, where each input data point is associated with a correct answer or label. The model learns to adjust its parameters to predict these labels as accurately as possible.
-
Proximal policy optimization — This method involves fine-tuning the LLM on a specific task using reinforcement learning from human feedback (RLHF).
-
Comparative ranking — This technique involves training the LLM to rank different outputs based on their relevance or quality, allowing the model to learn to generate more relevant and high-quality outputs.
SFT Method | Description | When to Use |
---|---|---|
LoRA (Low-Rank Adaptation) | Reduces trainable parameters. | Use when you need efficient fine-tuning with limited computational resources. |
QLoRA (Quantized LoRA) | Memory-efficient variant of LoRA. | Use when memory constraints are a concern and you need to fine-tune large models. |
Hyperparameter Tuning | Manually adjusts learning rate and batch size. | Use when you need to optimize model performance through parameter adjustments. |
Transfer Learning | Fine-tunes on a specific task. | Use when you have labeled data for a new task and want to leverage pre-trained knowledge. |
Multi-task Learning | Trains on multiple tasks simultaneously. | Use when you want the model to learn shared representations across tasks. |
Few-shot Learning | Trains on a small number of labeled examples. | Use when labeled data is scarce and you want to leverage the model's pre-existing knowledge. |
Task-specific Fine-Tuning | Trains the entire model on task-specific data. | Use when you need to adapt all model layers for a specific task. |
Reward Modeling | Trains on a labeled dataset with correct answers. | Use when you need the model to predict labels accurately based on rewards. |
Proximal Policy Optimization | Fine-tunes using reinforcement learning from human feedback. | Use when the task involves learning from human preference to improve performance. |
Comparative Ranking | Trains the model to rank outputs based on relevance. | Use when you need the model to generate high-quality, relevant outputs. |
These fine-tuning techniques help adapt pre-trained LLMs to specific tasks and domains, improving their performance and making them more suitable for various applications.
What are some common issues with supervised fine-tuning?
Some common issues with supervised fine-tuning of Large Language Models (LLMs) include:
-
Overfitting — Overfitting occurs when the model becomes too specific to the training data, leading to suboptimal generalization on unseen data. This is a common issue in machine learning and can also arise during the fine-tuning process of LLMs.
-
Hyperparameter tuning — Selecting inappropriate hyperparameters can lead to slow convergence, poor generalization, or even unstable training. Mastering hyperparameter tuning is a critical challenge that can be a burden on time and resources.
-
Data quality issues — Fine-tuning LLMs is only as good as the quality of the data provided. Insufficient knowledge from the model or data source can lead to poor fine-tuning results.
-
Catastrophic forgetting — This phenomenon occurs when fine-tuning a pre-trained model causes it to forget previously learned knowledge, leading to instability in the fine-tuning process.
-
Inconsistent performance — Fine-tuning LLMs can sometimes result in inconsistent performance on edge cases or inability to fit enough few-shot prompts in the context window to steer the model.
Addressing the common issues with supervised fine-tuning of Large Language Models (LLMs) involves several strategies. Hyperparameter tuning can be automated using techniques such as grid search or Bayesian optimization, which efficiently explore the hyperparameter space to identify optimal configurations. Overfitting and catastrophic forgetting can be combated by incorporating techniques like knowledge distillation. Ensuring the quality and relevance of data for the specific task or use case is crucial. Lastly, the fine-tuning process should be monitored and evaluated through continuous error analysis and iterative improvement.
FAQs
What is the difference between supervised fine-tuning and unsupervised fine-tuning?
Supervised fine-tuning refines a pre-trained model with labeled data, providing explicit examples of input-output pairs to guide the learning process. This targeted approach often yields higher accuracy but requires more computational resources due to the complexity of the loss function minimization involved.
In contrast, unsupervised fine-tuning does not use labeled data, instead relying on the model's intrinsic ability to discern patterns and structure within the data. This method is computationally less demanding but may not reach the performance levels of supervised methods due to the lack of direct feedback.
The decision to use supervised or unsupervised fine-tuning hinges on the task at hand, the availability of labeled data, computational budget, and performance requirements. Supervised fine-tuning is typically the go-to method for maximum precision, while unsupervised fine-tuning is an option when resources are constrained or labeled data is not accessible.
How does supervised fine-tuning differ from transfer learning?
While both involve adapting a pre-trained model to a new task, supervised fine-tuning typically requires labeled data and focuses on adjusting the model's weights on that data. Transfer learning can also involve using a pre-trained model as a starting point and then training it on a new dataset, which may not necessarily be labeled.
Can supervised fine-tuning be applied to any LLM?
In theory, yes. However, the effectiveness of fine-tuning can depend on factors such as the size of the LLM, the quality and quantity of the fine-tuning data, and the similarity between the pre-training tasks and the target task.
What are some best practices to avoid overfitting during supervised fine-tuning?
Best practices include using techniques like dropout, early stopping, and data augmentation, as well as carefully selecting the hyperparameters and employing regularization methods. It's also important to use a validation set to monitor the model's performance and prevent it from learning noise in the training data.
How do you measure the success of supervised fine-tuning?
The success of supervised fine-tuning is gauged through a variety of evaluation metrics that assess the model's performance on a test set it has not encountered during training. While accuracy, precision, recall, and F1 score are standard metrics, the choice of LLM evaluation depends on the fine-tuning objectives and the nature of the task.