MT-Bench (Multi-turn Benchmark)
by Stephen M. Walker II, Co-Founder / CEO
What is MT-Bench?
MT-Bench is a challenging multi-turn benchmark that measures the ability of large language models (LLMs) to engage in coherent, informative, and engaging conversations. It is designed to assess the conversation flow and instruction-following capabilities of LLMs, making it a valuable tool for evaluating their performance in understanding and responding to user queries.
In addition to the benchmark score, you can participate in the Elo arena and provide human feedback. Preference feedback is used to generate the Elo leaderboard. As of March 2024, the leaderboard includes models such as Anthropic Claude 3, OpenAI GPT-4 Turbo, Mistral Medium and Large, Google Gemini Pro, Mixtral-8x7b, and Tulu 2 among others.
The leaderboard is updated regularly (last updated March 26, 2024), providing a dynamic view of the best LLMs in the field.
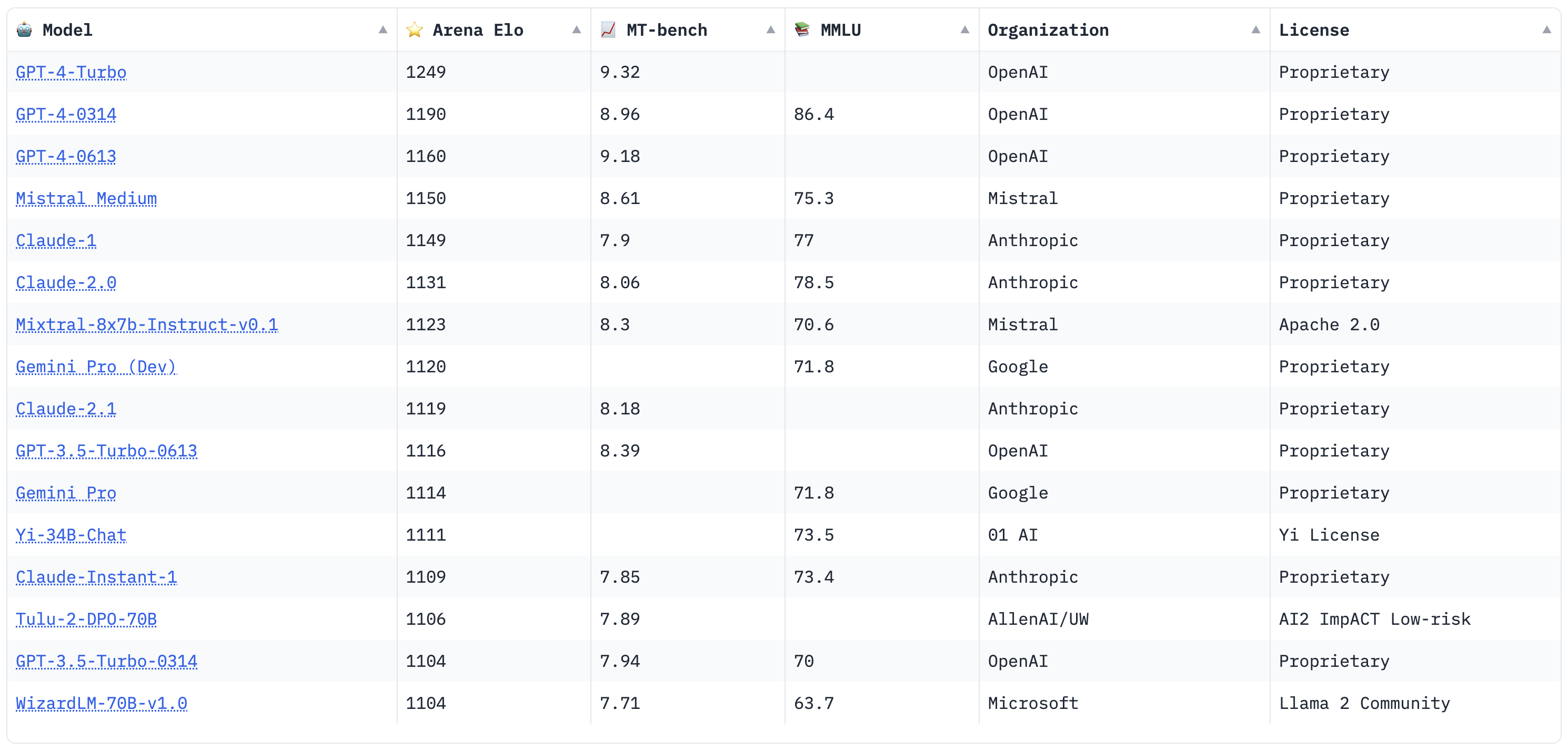
It's important to note that the Elo score reflects a model's performance on a comparative single response rather than a multi-turn conversation. This distinction is crucial because while some models may generate impressive initial responses, their performance can diminish more rapidly over multiple exchanges compared to others.
Key features of the MT Bench Leaderboard include:
- Challenging Multi-Turn Benchmark — The MT-Bench incorporates challenging follow-up questions as part of its design, ensuring that models demonstrate a deep understanding of the task at hand.
- Three Metrics — The leaderboard uses three metrics for evaluation: Chatbot Arena Elo, based on 200k+ anonymous votes from Chatbot Arena using the Elo rating system; MT-Bench score, based on a challenging multi-turn benchmark and GPT-4 grading; and MMLU, a widely adopted benchmark.
- Regular Updates — The leaderboard is updated regularly, providing a constantly evolving view of the latest LLM performance.
Triangulating relative model performance with MT-Bench and AlpacaEval provides the best benchmark for general performance from both human preference and LLM-as-judge perspectives. While performance on individual use cases may vary between models, these two benchmarks offer the most reliable standard.
MT-Bench Leaderboard (March 2024)
Model | Arena Elo rating | MT-bench (score) | MMLU | License |
---|---|---|---|---|
Claude 3 Opus | 1253 (+20) | 9.45 | 87.1 | Proprietary |
GPT-4-1106-preview | 1251 (0) | 9.40 | — | Proprietary |
GPT-4-0125-preview | 1248 (-3) | 9.38 | — | Proprietary |
Bard (Gemini Pro) | 1203 (0) | 9.18 | — | Proprietary |
Claude 3 Sonnet | 1198 (+18) | 9.22 | 87.0 | Proprietary |
GPT-4-0314 | 1185 (0) | 8.96 | 86.4 | Proprietary |
Claude 3 Haiku | 1179 | 9.10 | 86.9 | Proprietary |
GPT-4-0613 | 1158 (-3) | 9.18 | — | Proprietary |
Mistral-Large-2402 | 1157 (+2) | 8.63 | 75.5 | Proprietary |
Qwen1.5-72B-Chat | 1148 (+1) | 8.62 | 77.6 | Qiawen License |
Claude-1 | 1146 (0) | 7.9 | 77 | Proprietary |
Mistral Medium | 1145 (-2) | 8.59 | 75.2 | Proprietary |
Starling-LM-7B-beta | 1127 (+0) | 8.45 | 70.9 | Apache 2.0 |
Claude-2.0 | 1126 (-1) | 8.05 | 78.4 | Proprietary |
Gemini Pro (Dev API) | 1125 (+7) | 8.25 | 72.0 | Proprietary |
Mistral-Next | 1122 (-2) | 8.40 | — | Proprietary |
Claude-2.1 | 1115 (+1) | 8.19 | — | Proprietary |
GPT-3.5-Turbo-0613 | 1114 (-1) | 8.40 | — | Proprietary |
Mixtral-8x7b-Instruct-v0.1 | 1114 (0) | 8.3 | 70.6 | Apache 2.0 |
Gemini Pro | 1110 (-2) | 8.20 | 71.9 | Proprietary |
Claude-Instant-1 | 1104 (-1) | 7.86 | 73.5 | Proprietary |
WizardLM-70B-v1.0 | 1102 (+0) | 7.72 | 63.8 | Llama 2 Community |
GPT-3.5-Turbo-0314 | 1102 (-1) | 7.95 | 70.1 | Proprietary |
Yi-34B-Chat | 1099 (-1) | 7.90 | 73.6 | Yi License |
Tulu-2-DPO-70B | 1097 (-2) | 7.91 | — | AI2 ImpACT |
GPT-3.5-Turbo-0125 | 1097 (0) | 7.94 | 70 | Proprietary |
As of the latest update on March 26, the MT Bench Leaderboard has been refreshed, introducing the Claude 3 Haiku model. The initial update in March saw the introduction of new models from Mistral, Anthropic, and Qwen, which significantly altered the rankings, notably causing a decline in the positions of Bard/Gemini Pro. The February update was marked by the addition of the Qwen model and a noticeable shift in scoring preferences towards GPT-4 Turbo variants. On February 2, the leaderboard was expanded to include the gpt-4-0125-preview and Gemini Pro through Bard Assistant. Earlier, on January 10, the experimental Mixtral Medium
model was introduced, outperforming all Anthropic models in the rankings.
The MT Bench Leaderboard, which is regularly updated, evaluates the leading Large Language Models (LLMs) including Claude 3, GPT-4 Turbo, Mistral Medium, Gemini Pro, Mixtral-8x7b, and Tulu 2, based on their performance across a variety of tasks. This ensures a current and comprehensive overview of the top competitors in the field as of March 2024.
How does MT-Bench work?
MT-Bench is a challenging multi-turn question set designed to evaluate the conversational and instruction-following ability of large language models (LLMs).
The benchmark consists of 80 high-quality, multi-turn questions tailored to assess conversation flow and instruction-following capabilities.
Some key aspects of MT-Bench include:
- Purpose — MT-Bench aims to evaluate the performance of LLMs in open-ended conversations, approximating human preferences.
- Methodology — The benchmark uses fastchat.llm_judge and the Arena Elo calculator, with MMLU based on InstructEval and Chain-of-Thought Hub.
- Leaderboard — A leaderboard is maintained to track the performance of various LLMs, such as GPT-4-turbo, Vicuna-33B, WizardLM-30B, and others.
- Challenges — MT-Bench incorporates challenging follow-up questions as part of its design, making it a rigorous test for LLMs.
For practical use, the MT Bench prompts are available through the Hugging Face datasets library, allowing developers and researchers to evaluate chat models using the benchmark.
The MT-Bench dataset contains expert-level pairwise human preferences for model responses generated by LLMs like GPT-4, GPT-3.5, Claud-v1, Vicuna-13B, Alpaca-13B, and LLaMA-13B.
The benchmark is used in conjunction with Chatbot Arena, a crowdsourced battle platform where users ask chatbots any question and vote for their preferred answer. Both benchmarks aim to use human preferences as the primary metric for evaluating LLMs.
What is the purpose of MT-Bench?
The Multi-Turn Bench addresses the shortcomings of traditional benchmarks that struggle to differentiate between human-aligned and non-aligned LLMs. MT Bench uses a challenging multi-turn question set to assess the conversational and instruction-following abilities of models, simulating real-world conversational scenarios for a dynamic performance assessment.
MT Bench LLM Eval aims to provide a comprehensive, objective, and scalable method for evaluating LLMs, particularly in chatbot applications, by addressing the limitations of traditional benchmarks and offering a more dynamic and explainable evaluation process.
This makes it ideal for evaluating chatbots, which are expected to manage complex, multi-turn conversations. A distinctive feature of MT Bench is its use of strong LLMs as judges, which offers scalability and explainability.
Automation and Explainability
The automation of the evaluation process through LLM judges allows for rapid and scalable assessments, particularly beneficial when evaluating a large number of models or conducting frequent evaluations.
Additionally, LLM judges provide not only scores but also explanations, offering interpretable outputs and valuable insights.
Limitations of LLM-as-a-Judge
However, it's crucial to acknowledge the limitations of LLM-as-a-judge, such as its inability to detect hallucinations and penalize LLM generated answers accordingly, and potential errors when grading math/reasoning questions.
What are some criticisms of MT-Bench?
Some criticisms of the MT Bench include:
-
Position, verbosity, and self-enhancement biases — The paper examines the usage and limitations of LLM-as-a-judge, which can be affected by position, verbosity, and self-enhancement biases, as well as limited reasoning ability.
-
Limited reasoning ability — The paper also discusses the limited reasoning ability of LLM-as-a-judge, which may not be able to fully understand and evaluate the complexities of certain tasks or questions.
Despite these criticisms, the paper proposes solutions to mitigate some of the limitations and demonstrates that strong LLM judges, like GPT-4, can match both controlled and crowdsourced achieving over 80% agreement, the same level of agreement between humans. The benchmark and traditional benchmarks complement each other by evaluating various aspects of LLM performance, providing a more comprehensive understanding of their capabilities and limitations.
What are some future directions for MT-Bench research?
Some future directions for MT-Bench research include:
-
Expansion of language pairs and tasks — Incorporating more languages, dialects, and task types will broaden the scope of machine translation evaluation. This could involve adding new languages or working with low-resource and endangered languages.
-
Exploration of multimodal and cross-lingual tasks — Expanding MT-Bench to include multimodal and cross-lingual tasks such as image captioning, visual question answering, and language understanding can further assess the capabilities of translation models in real-world scenarios.
-
Inclusion of newer metrics and evaluation methods — As new metrics are developed to evaluate translation quality, incorporating these into MT-Bench will provide a more comprehensive assessment of machine translation systems. This could involve developing metrics for evaluating aspects such as fluency, coherence, and naturalness in translations.
-
Incorporation of real-world data — Utilizing authentic data from various domains, such as e-commerce, healthcare, or social media, can better reflect the real-world scenarios where machine translation models will be employed. This would also involve addressing challenges like handling noisy and incomplete data.
-
Improving benchmarking tools and methodologies — Developing advanced methods for preprocessing, postprocessing, and managing evaluation results can facilitate more accurate and reliable comparisons between different models and approaches.
-
Promoting collaboration and sharing of resources — Encouraging researchers to contribute datasets, models, metrics, and other resources to MT-Bench will promote a collaborative environment that fosters innovation and improves the quality of machine translation research overall.
Judging LLM-as-a-Judge
The paper "Judging LLM-as-a-Judge with MT-Bench and Chatbot Arena" explores the use of Large Language Models (LLMs) as judges to evaluate other LLMs, particularly in the context of chat assistants. The authors identify the challenges of evaluating LLMs due to their broad capabilities and propose using strong LLMs as judges to evaluate these models on more open-ended questions.
The paper introduces two benchmarks: MT-Bench, a multi-turn question set, and Chatbot Arena, a crowdsourced battle platform. These benchmarks are used to verify the agreement between LLM judges and human preferences. The results reveal that strong LLM judges like GPT-4 can match both controlled and crowdsourced human preferences, achieving over 80% agreement, which is the same level of agreement between humans.
The authors also examine the usage and limitations of LLM-as-a-judge, including position bias, verbosity bias, self-enhancement bias, and limited reasoning ability. They propose solutions to mitigate some of these biases. The paper concludes that LLM-as-a-judge is a scalable and explainable way to approximate human preferences, which are otherwise very expensive to obtain.
The paper's data, including the MT-bench questions, 3K expert votes, and 30K conversations with human preferences, are publicly available.
What does MT stand for?
MT-Bench stands for "Multi-Turn Benchmark," in which the "MT" is often mistakenly thought to refer to machine translation.
Looking at you Claude...
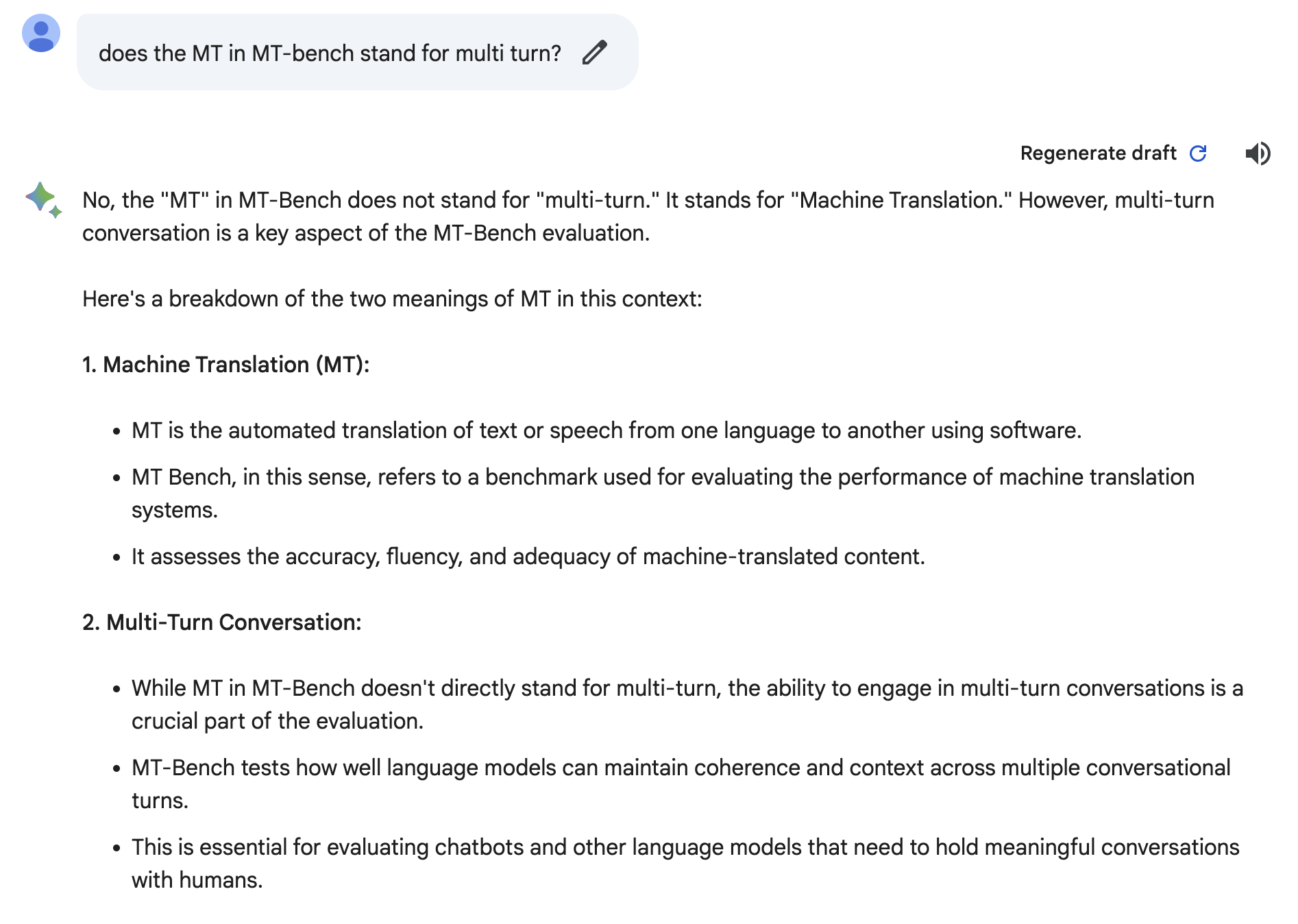