MMMU: Massive Multi-discipline Multimodal Understanding and Reasoning Benchmark
by Stephen M. Walker II, Co-Founder / CEO
What is the MMMU (Massive Multi-discipline Multimodal Understanding and Reasoning) Benchmark?
The MMMU benchmark, which stands for Massive Multi-discipline Multimodal Understanding and Reasoning, is a new benchmark designed to evaluate the capabilities of multimodal models on tasks that require college-level subject knowledge and expert-level reasoning across multiple disciplines.
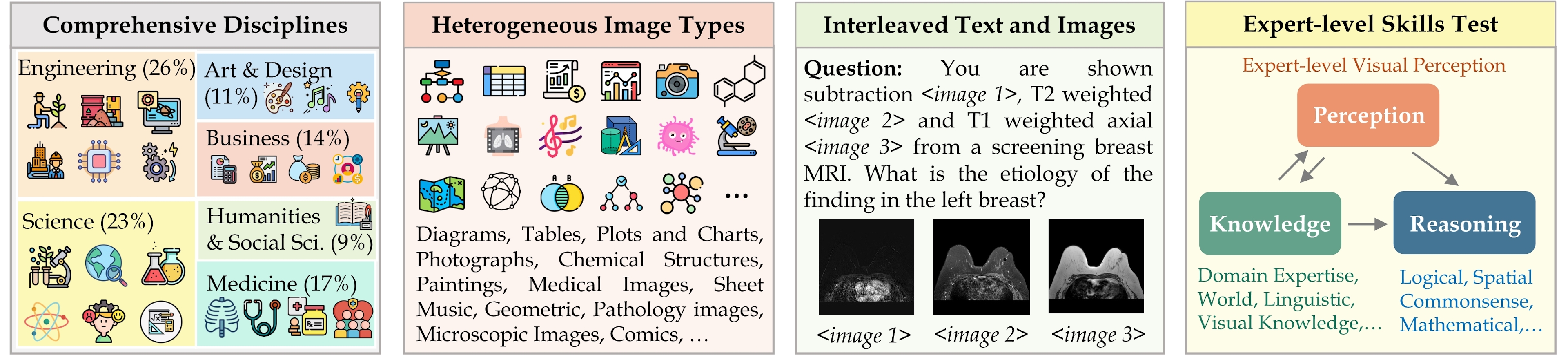
It covers six core disciplines: Art & Design, Business, Health & Medicine, Science, Humanities & Social Science, and Technology & Engineering, and includes over 183 subfields. The benchmark includes a variety of image formats such as diagrams, tables, charts, chemical structures, photographs, paintings, geometric shapes, and musical scores, among others.
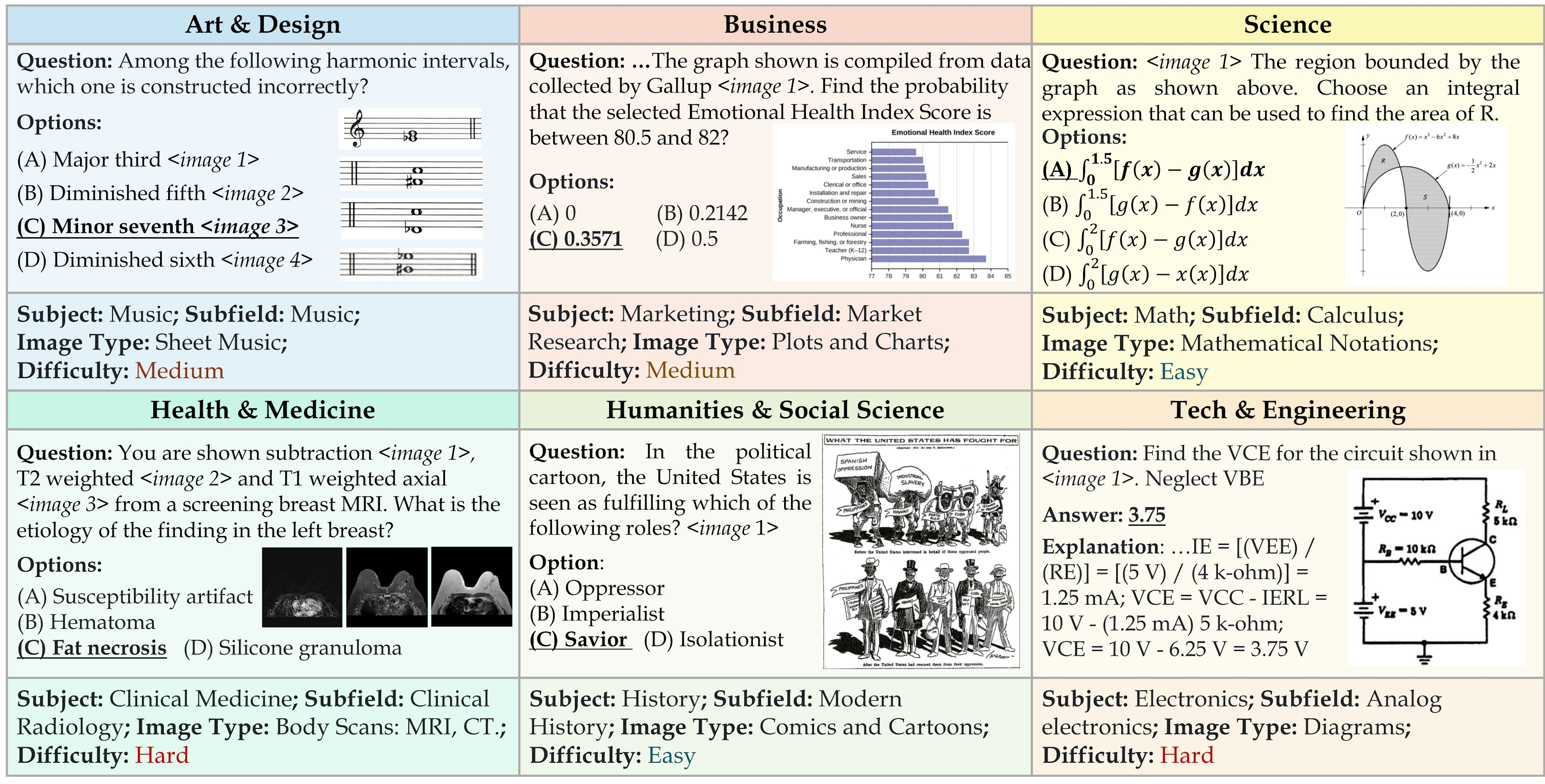
The MMMU benchmark, designed to surpass traditional benchmarks limited to everyday knowledge, assesses advanced AI capabilities through expert-level questions.
These questions are meticulously sourced from university textbooks and lecture materials by a dedicated team of 50 students, including co-authors. The benchmark's structure includes a few-shot development set, a validation set, and a comprehensive test set with 10.5K questions.
The MMMU benchmark is considered a valuable tool for evaluating the capabilities of large language models (LLMs) and is seen as a step towards the advancement of Artificial General Intelligence (AGI). It has been used to evaluate various models, including both closed- and open-source models, under a zero-shot setting. Models like GPT-4V and others have been evaluated on this benchmark, with GPT-4V achieving a score of 55.7% accuracy. The benchmark is relatively new, having been introduced in November 2023, and is already influencing the field of AI.
Current Leaderboard
As of the latest update, the leaderboard is currently led by GPT-4o with an overall score of 69.1, followed closely by Claude 3.5 Sonnet with a score of 68.3, and Gemini 1.5 Pro with a score of 62.2. Claude 3 Opus and Gemini 1.0 Ultra are tied for the next position with a score of 59.4. Notably, GPT-4 Vision is also a strong contender with a score of 56.8, excelling in several disciplines.
In real-world tests, GPT-4o performs better at tasks describing screenshots or analyzing tabular data in images. Gemini Ultra is much better than Pro at articulating nuanced differences in images. GPT-4o is better at spotting specific details in images. Anthropic Claude 3.5 Sonnet is superior to all in its ability to correctly label large grids of information (30x30), but struggles when visuals are on dark backgrounds.
The MMMU benchmark's current leaderboard is led by GPT-4o with an overall score of 69.1, followed closely by Claude 3.5 Sonnet with a score of 68.3, and Gemini 1.5 Pro with a score of 62.2. Notably, GPT-4 Vision excels in several disciplines with an overall score of 56.8%, performing exceptionally well in Art & Design (65.3%), Business (64.3%), Health & Medicine (63.5%), and Humanities & Social Sciences (76.3%), but lagging in Science (54.7%) and Tech & Engineering (41.7%). Among open-source models, Qwen-VL-MAX* leads with an overall score of 46.8%. This benchmark evaluates multimodal models across six disciplines using 11.5K questions from college-level materials, aiming to push the limits of expert-level reasoning in AI.
Model | Overall | Art & Design | Business | Science | Health & Medicine | Human. & Social Sci. | Tech & Eng. |
---|---|---|---|---|---|---|---|
GPT-4o | 69.1 | - | - | - | - | - | - |
Claude 3.5 Sonnet | 68.3 | - | - | - | - | - | - |
Gemini 1.5 Pro | 62.2 | - | - | - | - | - | - |
Claude 3 Opus | 59.4 | - | - | - | - | - | - |
Gemini 1.0 Ultra | 59.4 | 70 | 56.7 | 48 | 67.3 | 78.3 | 47.1 |
GPT-4 Vision | 56.8 | 65.3 | 64.3 | 54.7 | 63.5 | 76.3 | 41.7 |
Gemini 1.5 Flash | 56.1 | - | - | - | - | - | - |
Claude 3 Sonnet | 53.1 | - | - | - | - | - | - |
Claude 3 Haiku | 50.2 | - | - | - | - | - | - |
Gemini 1.0 Pro | 47.9 | - | - | - | - | - | - |
Along with a range of open source models.
Model | Overall | Art & Design | Business | Science | Health & Medicine | Human. & Social Sci. | Tech & Eng. |
---|---|---|---|---|---|---|---|
Qwen-VL-MAX* | 46.8 | 64.2 | 39.8 | 36.3 | 52.5 | 70.4 | 40.7 |
Yi-VL-34B* | 41.6 | 56.1 | 33.3 | 32.9 | 45.9 | 66.5 | 36.0 |
Qwen-VL-PLUS* | 40.8 | 59.9 | 34.5 | 32.8 | 43.7 | 65.5 | 32.9 |
Marco-VL* | 40.4 | 56.5 | 31.0 | 31.0 | 46.9 | 66.5 | 33.8 |
InternLM-XComposer2-VL* | 38.2 | 56.8 | 32.8 | 30.1 | 39.8 | 60.7 | 31.8 |
Yi-VL-6B* | 37.8 | 53.4 | 30.3 | 30.0 | 39.3 | 58.5 | 34.1 |
InfiMM-Zephyr-7B* | 35.5 | 50.0 | 29.6 | 28.2 | 37.5 | 54.6 | 31.1 |
InternVL-Chat-V1.1* | 35.3 | 53.7 | 31.7 | 28.2 | 36.5 | 56.4 | 28.0 |
SVIT* | 34.1 | 48.9 | 28.0 | 26.8 | 35.5 | 50.9 | 30.7 |
Emu2-Chat* | 34.1 | 50.6 | 27.7 | 28.0 | 32.4 | 50.3 | 31.3 |
BLIP-2 FLAN-T5-XXL | 34.0 | 49.2 | 28.6 | 27.3 | 33.7 | 51.5 | 30.4 |
InstructBLIP-T5-XXL | 33.8 | 48.5 | 30.6 | 27.6 | 33.6 | 49.8 | 29.4 |
LLaVA-1.5-13B | 33.6 | 49.8 | 28.2 | 25.9 | 34.9 | 54.7 | 28.3 |
Google's Gemini Ultra set a new standard on the MMMU benchmark with a 59.4% overall accuracy, outperforming models like GPT-4V. It demonstrated strong capabilities in Business (62.7%), Health and Medicine (71.3%), Humanities and Social Science (78.3%), and Technology and Engineering (53.0%). While MMMU is a rigorous test of multimodal understanding and reasoning, it is not without limitations. It may not encompass all aspects of Expert AGI, as it primarily focuses on college-level knowledge. Nevertheless, a high score on MMMU is considered indicative of a model's proficiency towards achieving Expert AGI.
How does MMMU work?
The MMMU benchmark operates by evaluating large multimodal models (LMMs) on their ability to perceive, understand, and reason across a wide range of disciplines and subfields using various image types. It is designed to measure three essential skills in LMMs: perception, knowledge, and reasoning.
The benchmark includes over 11.5K questions that span 30 subjects and 183 subfields, comprising 30 highly heterogeneous image types such as diagrams, tables, charts, chemical structures, photographs, paintings, geometric shapes, and musical scores.
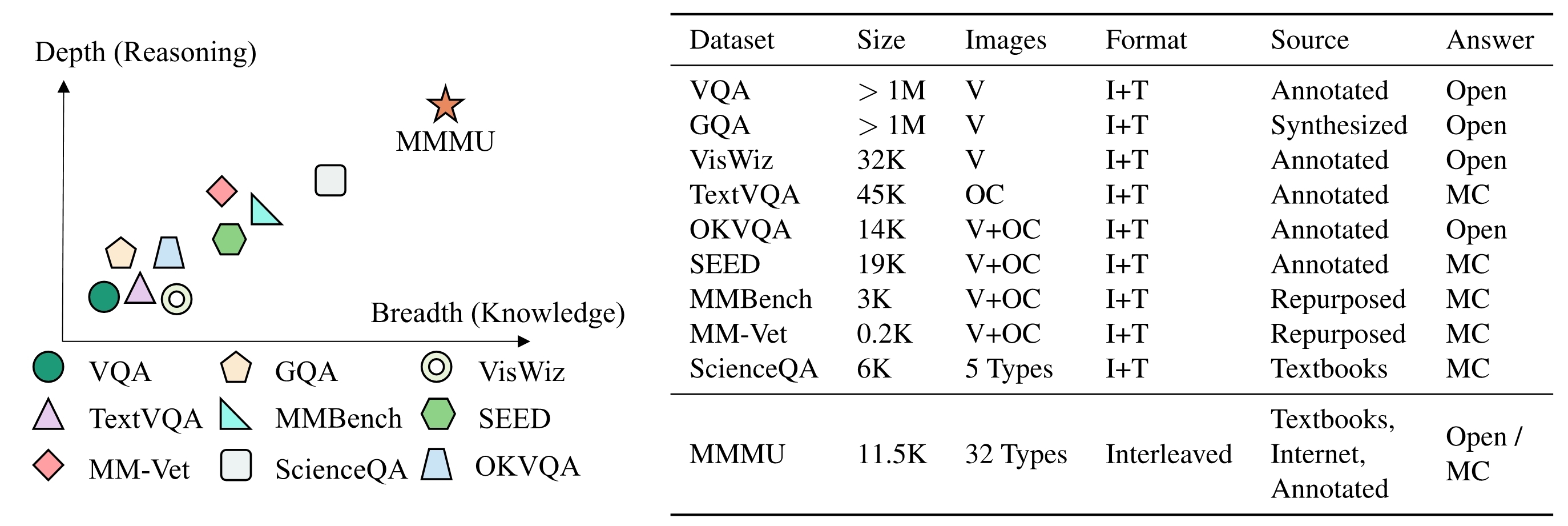
The MMMU benchmark assesses models in a zero-shot setting, meaning that the models are tested on their ability to generate answers without any prior specific training or fine-tuning on the benchmark's tasks. This approach is intended to gauge the models' innate capabilities.
The benchmark is divided into a few-shot development set, a validation set, and a test set, with the test set comprising 10.5K questions. The few-shot development set includes 5 questions per subject, and the validation set contains approximately 900 questions.
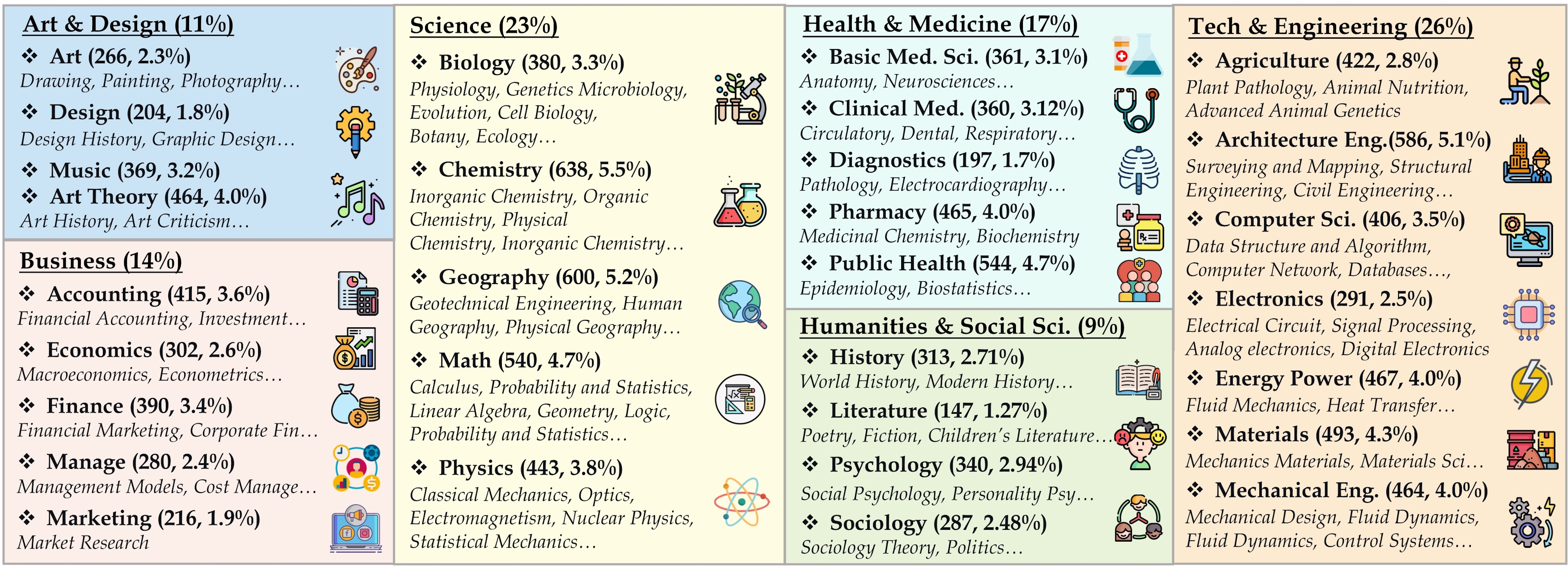
Models are evaluated on their performance across the six core disciplines, and the results are presented with the best-performing model in each category highlighted in bold and the second best underlined. The benchmark's comprehensiveness and focus on college-level subject knowledge and expert-level reasoning make it a valuable tool for advancing the development of artificial general intelligence (AGI).
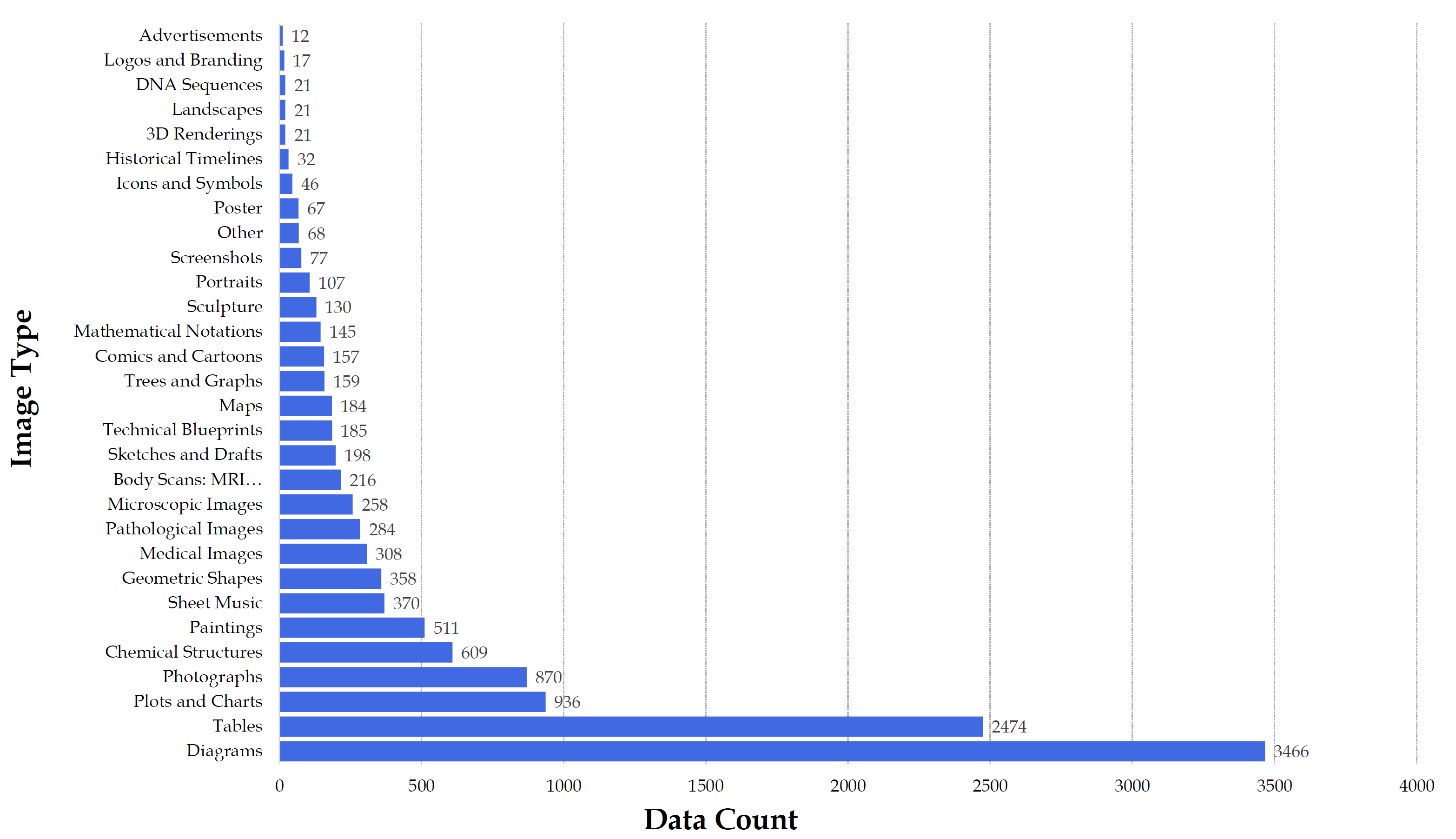
The MMMU benchmark is also used to identify specific areas where models need improvement. For example, an analysis of mispredicted cases by GPT-4Vision showed that errors could be attributed to flaws in visual perception, lack of domain knowledge, or reasoning process flaws. This detailed feedback helps guide further research and development of multimodal models.
When to use MMMU
The MMMU benchmark, designed for evaluating multimodal models, is pivotal for assessing large language models' (LLMs) understanding and reasoning across diverse disciplines. It focuses on college-level knowledge within six core disciplines—Art & Design, Business, Science, Health & Medicine, Humanities & Social Science, and Tech & Engineering—encompassing 30 subjects and 183 subfields. The benchmark's 30 image types, including charts, diagrams, and chemical structures, are sourced from academic textbooks and lectures.
MMMU stands out by testing expert-level perception and reasoning, requiring domain-specific knowledge. It challenges models to provide precise answers without prior fine-tuning or few-shot learning, aiming to simulate expert tasks. For example, Google's Gemini Ultra model set a new benchmark record with a 59.4% accuracy, surpassing GPT-4V's 56%. These results highlight the benchmark's role in identifying areas for AI improvement and guiding future research.
When considering the use of MMMU, it's important to recognize its role in pushing the boundaries of AI capabilities. It is particularly useful for gauging a model's proficiency in expert-level reasoning and multimodal understanding, which are critical for advancements towards Artificial General Intelligence (AGI).
Limitations of MMMU
The Massive Multi-discipline Multimodal Understanding and Reasoning Benchmark (MMMU) is a dataset designed to evaluate the capabilities of large language models (LLMs) and multimodal models in understanding and reasoning across a wide range of disciplines and modalities. However, MMMU has several limitations:
-
Limited Data Availability: MMMU relies on a limited dataset of questions and images, which could affect the generalizability of its results and raise the potential for overfitting. Expanding the dataset with more diverse and representative data is necessary for improving the benchmark.
-
Lack of Explanation: The benchmark provides limited insights into the reasoning processes of LLMs, making it difficult to understand why LLMs make certain mistakes and how their performance can be improved.
-
Comprehensiveness: While MMMU's comprehensiveness is a strength, it also presents a challenge. The benchmark covers six core disciplines and over 30 different image formats, which can be difficult for models to interpret and understand, especially when it comes to complex scientific concepts and notations.
-
Performance Gaps: Even advanced models like GPT-4V(ision) and Gemini Ultra only achieve accuracies of 56% and 59% respectively, indicating significant room for improvement in the field.
-
Manual Curation Process: The manual curation process used to create MMMU may carry biases, and the focus on college-level subjects might not fully test the capabilities required for Expert AGI.
-
Model Performance Variability: Performance varies across disciplines, with better results in visually simpler fields compared to more complex fields like Science and Engineering.
-
Need for Advanced Joint Interpretation: Additional features like OCR and captioning do not substantially enhance performance, highlighting the need for more advanced joint interpretation of images and text.
To address these limitations, future work on MMMU includes plans to incorporate human evaluations and to expand the dataset to ensure it captures a broader and deeper range of knowledge and reasoning skills.