Kardashev Gradient
by Stephen M. Walker II, Co-Founder / CEO
What is the Kardashev Gradient?
The Kardashev Gradient is a concept that combines the Kardashev Scale, a method for measuring a civilization's level of technological advancement based on energy consumption, with the concept of network motifs, which are recurring patterns of connectivity within a complex network. In the context of AI and Large Language Models (LLMs), the Kardashev Gradient can be used to understand and predict the trajectory of AI development and its potential impact on society.
The Kardashev Scale, proposed by Soviet astronomer Nikolai Kardashev, describes three levels of civilization based on energy consumption:
- Type I Civilizations — Also known as planetary civilizations, these can capture and harness all available energy on their planet (10^16 watts).
- Type II Civilizations — Also called stellar civilizations, these can harness the total energy of their planet (10^26 watts).
- Type III Civilizations — Also called galactic civilizations, these can harness all available energy from their entire host galaxy (10^36 watts).
Projecting from present time to the future indicates an extraordinary paradigm shift in energy consumption patterns, signaling a civilization on the brink of transitioning to a Type I status on the Kardashev scale. The exponential increase in energy use, particularly from renewable sources, suggests that by 2400, our civilization will have mastered the harnessing of planetary resources to a degree that significantly surpasses our current capabilities.
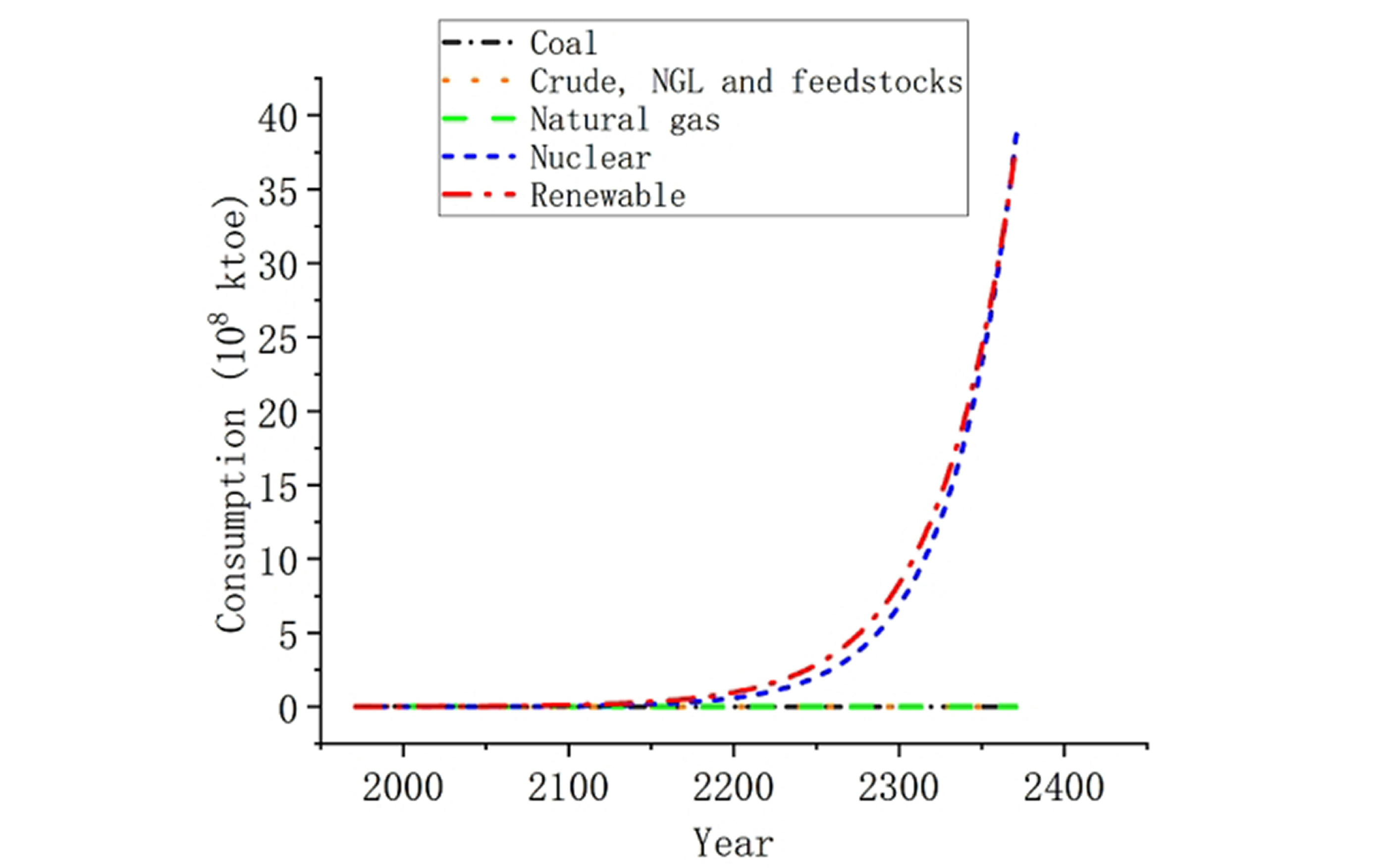
If this trend holds true, it would represent a monumental leap in technological advancement, potentially bringing about an era of unprecedented energy abundance and a transformation in the way this civilization sustains itself and interacts with its environment.
In the context of AI and LLMs, the Kardashev Gradient can be used to analyze the advancement of AI technologies and their potential impact on energy consumption and civilization development. For example, the Kardashev scale has been applied to forecast the progression of human civilization through 2060, using machine learning approaches to improve previous projections. This allows for a more accurate understanding of the relationship between energy consumption, AI development, and the potential for humanity to ascend to higher levels of civilization.
The Kardashev Gradient is a powerful tool for understanding the trajectory of AI development and its potential impact on society, by combining the Kardashev Scale with the concept of network motifs. This framework allows for a more comprehensive analysis of the relationship between energy consumption, AI advancements, and the development of civilizations.
What is Kardashev Scale?
The Kardashev Scale, proposed by Soviet astronomer Nikolai Kardashev in 1964, is a method of measuring a civilization's level of technological advancement based on the amount of energy it is capable of using.
The scale has since been expanded and refined by other scientists, including Carl Sagan, who proposed a continuous function for the Kardashev Scale to allow for finer gradations between the types. As of 2018, human civilization was estimated to be at approximately 0.728 on this scale.
The concept of the Kardashev Gradient involves understanding the potential stages of AI development, from AI that can perform specific tasks (Type I), to AI that can perform any intellectual task that a human being can do (Type II), and finally to AI that can control or manipulate the entire universe (Type III).
The Kardashev Gradient is used in a variety of discussions and thought experiments in AI, including those related to the potential risks and benefits of advanced AI, the ethical considerations of AI development, and the future of humanity in an AI-dominated world.
How does the Kardashev Gradient work in AI?
The application of the Kardashev Gradient in AI involves several considerations:
-
Understanding the Stages — The first step is understanding the potential stages of AI development, as outlined by the Kardashev Scale. This can provide a roadmap for AI progress and help us anticipate the challenges and opportunities at each stage.
-
Assessing Current AI Technologies — The next step is assessing where current AI technologies fall on the Kardashev Gradient. This can help us understand the current state of AI development and its potential trajectory.
-
Anticipating Future Developments — Based on our understanding of the Kardashev Gradient and the current state of AI, we can begin to anticipate future developments in AI. This can include predicting the potential risks and benefits of advanced AI, as well as the ethical considerations and societal impacts of such technologies.
-
Guiding AI Development — The Kardashev Gradient can also serve as a guide for AI development, helping us make informed decisions about the direction of AI research and development.
What are the benefits of the Kardashev Gradient in AI?
The Kardashev Gradient offers several benefits in the context of AI:
-
Framework for Understanding AI Progress — The Kardashev Gradient provides a framework for understanding and predicting the trajectory of AI development. This can help us anticipate the challenges and opportunities that advanced AI technologies might bring.
-
Guidance for AI Development — The Kardashev Gradient can serve as a guide for AI development, helping us make informed decisions about the direction of AI research and development.
-
Tool for Anticipating Risks and Benefits — The Kardashev Gradient can help us anticipate the potential risks and benefits of advanced AI. This can inform discussions about AI ethics and the societal impact of AI technologies.
-
Aid in Future Planning — By providing a roadmap for AI progress, the Kardashev Gradient can aid in future planning, helping us prepare for a future in which AI plays an increasingly significant role.
What are some applications of the Kardashev Gradient in AI?
The Kardashev Gradient can be applied in a variety of ways in AI, including:
-
AI Ethics Discussions — The Kardashev Gradient can inform discussions about AI ethics, helping us anticipate the ethical considerations that might arise as AI technologies advance.
-
AI Development Planning — The Kardashev Gradient can guide AI development planning, helping us make informed decisions about the direction of AI research and development.
-
Risk Assessment — The Kardashev Gradient can aid in risk assessment, helping us anticipate the potential risks of advanced AI technologies.
-
Future Planning — By providing a roadmap for AI progress, the Kardashev Gradient can aid in future planning, helping us prepare for a future in which AI plays an increasingly significant role.
These are just a few examples of the many applications of the Kardashev Gradient in AI.