LangChain
by Stephen M. Walker II, Co-Founder / CEO
LangChain
LangChain is an open-source framework designed to simplify the creation of applications using large language models (LLMs). It provides a standard interface for chains, integrations with other tools, and end-to-end chains for common applications. LangChain allows developers to build applications based on combined LLMs, such as GPT-4, with external sources of computation and data. The framework is written in Python and JavaScript and supports various language models, including those from OpenAI, HuggingFace, and others.
LangChain's core components include:
- Components: Modular building blocks that are easy to use for building powerful applications, such as LLM wrappers, prompt templates, and indexes for relevant information retrieval.
- Chains: Allow developers to combine multiple components to solve specific tasks, making the implementation of complex applications more modular and easier to debug and maintain.
- Agents: Enable LLMs to interact with their environment, such as using external APIs to perform specific actions.
LangChain can be used to build a wide range of LLM-powered applications, such as chatbots, question-answering systems, text summarization, code analysis, and more.
Monitoring LLM applications
Due to the stochastic nature of LLMs and LangChain agents, monitoring LLM apps is crucial to systematically drive improvements.
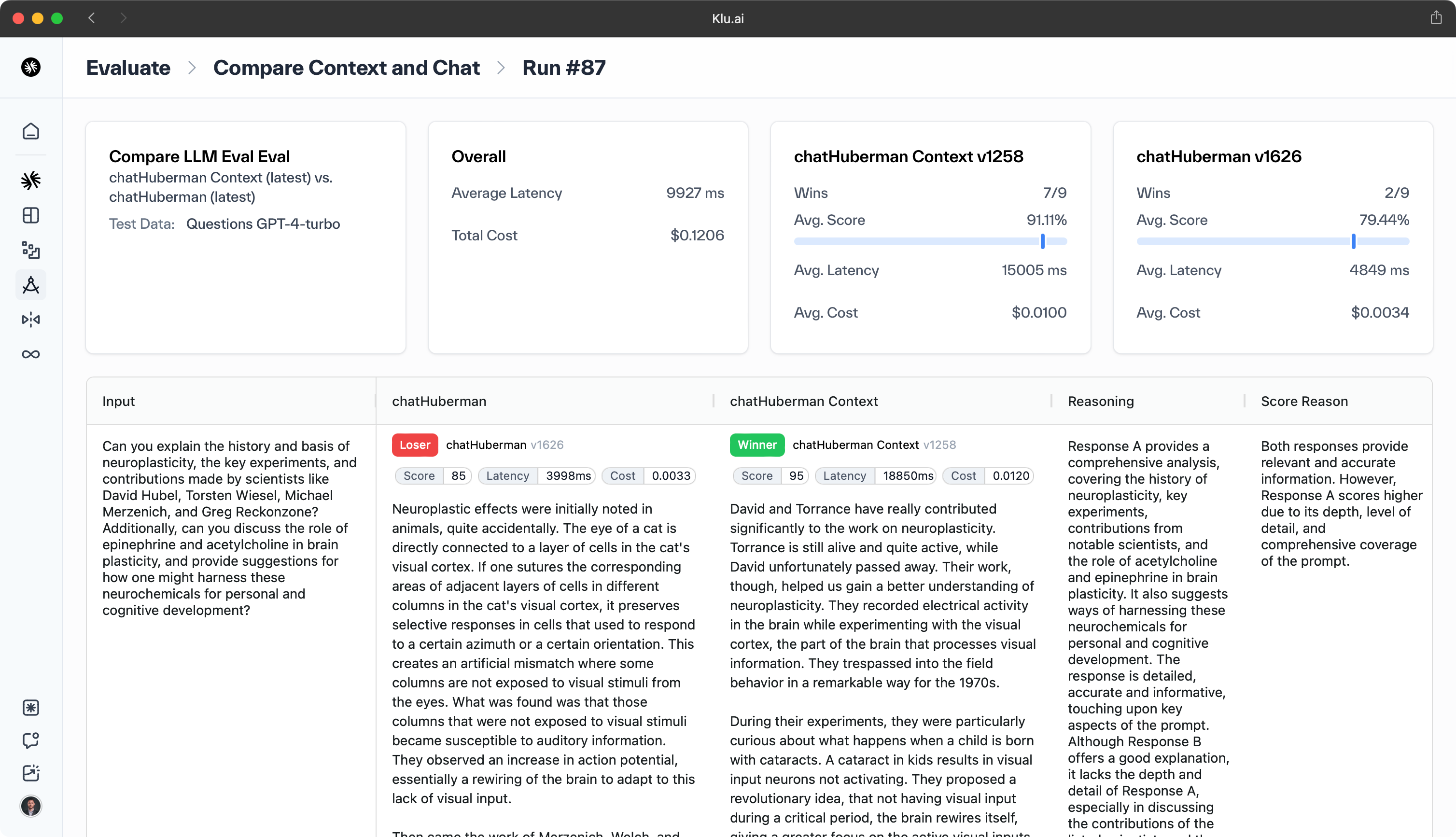
Klu.ai enhances LangChain developers' capabilities by offering advanced observability and monitoring tools, ensuring that applications are not only robust but also transparent in their operations.
These tools provide superior insights into agent performance and behavior, facilitating a more efficient debugging and development process.
Langchain expression language
The LangChain Expression Language (LCEL) is a declarative framework designed to simplify the composition of chains for working with large language models (LLMs). It allows developers to build chains that support synchronous, asynchronous, and streaming interactions with LLMs. LCEL is integrated with tools like LangSmith for tracing and LangServe for deployment, ensuring observability and ease of deployment for complex chains.
LCEL provides several benefits:
- Streaming Support — LCEL chains offer time-to-first-token efficiency, allowing intermediate results to be streamed, which is useful for user feedback or debugging.
- Input and Output Schemas — Chains built with LCEL automatically include Pydantic and JSONSchema for input and output validation, which is a core part of LangServe.
- Seamless Integration — LCEL integrates with LangSmith for tracing and LangServe for deployment, making it easier to understand chain operations and deploy them.
The language is designed to be easy to learn, with a focus on rapid prototyping and production readiness. It abstracts complex Python concepts into a minimalist code layer, supporting advanced features like parallel execution and easy integration with other LangChain components.
LCEL's syntax allows for easy chaining of components using pipe operations, and it abstracts away the optimization of LLM calls, handling batch, stream, and async APIs. It also provides a clear interface for modifying prompts, which are central to customizing the behavior of LLMs.
For practical examples and tutorials on using LCEL, there are resources available on YouTube, as well as detailed guides and documentation on the official LangChain website. These resources can help you get started with LCEL and leverage its capabilities for your generative AI projects.
Support for language models
LangChain is an open-source framework designed to simplify the development of applications powered by LLMs such as OpenAI's GPT-4 or Google's PaLM. It provides abstractions for common use cases and supports both JavaScript and Python.
LangChain is organized into six modules, each designed to manage a different aspect of the interaction with LLMs:
- Models — This module allows you to instantiate and use different LLMs.
- Prompts — This module manages prompts, which are the means of interacting with the model to obtain an output. It includes functionalities for creating reusable prompt templates.
- Indexes — This module helps in combining the best models with your textual data to add context or explain something to the model.
- Chains — This module allows the integration of other tools. For example, one call can be a composed chain with a specific purpose.
- Conversational Memory — LangChain incorporates memory modules that enable the management and alteration of past chat, a key feature for chatbots that need to recall previous interactions.
- Intelligent Agents — This module equips LangChain with the ability to create intelligent agents.
These modules target specific development needs, making LangChain a comprehensive toolkit for creating advanced language model applications.
LangChain provides an LLM class that allows us to interact with different language model providers, such as OpenAI and Hugging Face. It also provides APIs with which developers can connect and query LLMs from their code.
LangChain's Chains and Agents features further the potential of applications that can be built using LangChain in just a few lines. For more complex tasks, it usually requires chaining multiple steps and/or models. The newer and recommended method for using Chains in LangChain is through the LangChain Expression Language (LCEL).
LangChain is under active development, and its use in production should be handled with care. It is supported by an active community, and organizations can use LangChain for free and receive support from other developers proficient in the framework.
LangChain's versatility and power are evident in its numerous real-world applications, which include Q&A systems, data analysis, code understanding, chatbots, and summarization. As technology advances, the potential of LangChain and AI-powered language modeling should continue to grow.
Integrating external data sources
LangChain is an open-source framework that allows developers to integrate large language models (LLMs) like GPT-4 with external data sources and APIs. This integration enables the creation of powerful AI applications that can extract information from custom data sources and interact with external APIs.
LangChain provides several tools and libraries to facilitate this integration:
-
LLM Wrappers — These are used to interact with LLMs and create prompt templates, build chains, and work with embeddings and vector stores.
-
Document Loaders — These tools load data as documents from a variety of sources, including proprietary sources that may require additional authentication.
-
Extraction Chains — These are used to extract structured data from unstructured text. For example, you can use the
create_extraction_chain
function to extract a desired schema using an OpenAI function call. -
API Chains — These allow the LLM to interact with external APIs, which can be useful for retrieving context for the LLM to utilize.
-
Database Integration — LangChain can be integrated with databases to facilitate natural language interactions. Users can ask questions or make requests in plain English, and LangChain translates these into the corresponding queries.
-
Tools for Interacting with Various Services — LangChain provides tools for interacting with a wide range of services, including Alpha Vantage, Apify, ArXiv, AWS Lambda, Bing Search, Google Cloud Text-to-Speech, Google Drive, Google Finance, and many more.
To utilize LangChain, install the required libraries and set up API keys for services like OpenAI and Pinecone. LangChain enables interaction with LLMs, creation of prompt templates, chain building, and management of embeddings and vector stores.
LangChain's versatility extends to extracting information from documents or databases, leveraging GPT-4 for actions such as drafting data-specific emails. Additionally, it supports the development of chatbots with advanced conversational memory, fostering richer and more context-aware user interactions.
FAQ
How does LC integrate models and machine learning to handle queries?
The library provides a generic interface that facilitates the integration of various language models into machine learning applications. This interface allows developers to craft prompt templates tailored to specific user queries. By utilizing these templates within the data augmented generation process, LangChain enhances the language model's ability to understand and respond to queries, making it a versatile tool for developers in the rapidly evolving field of machine learning.
How can I customize existing chains to handle user input and integrate with an external data source?
The unified developer platform provides a robust framework that allows developers to customize existing chains to handle user input effectively. By leveraging the libraries and crafting a prompt template, developers can create applications that seamlessly integrate with external data sources. This integration empowers LangChain to process user queries with greater context and accuracy, enhancing the overall user experience within the language model ecosystem.
How can I ensure my LangChain app effectively processes the user's input and integrates with external data sources?
To ship LLM apps that are responsive to the user's input and capable of integrating with external data sources, it is essential to utilize the standard interface provided by LC libraries. This interface allows developers to customize existing chains within the rapidly developing field of AI and language models. By doing so, you can create applications that not only understand the context of user queries but also fetch relevant data from external APIs or databases, ensuring a seamless and efficient user experience.
How can data scientists leverage LangChain for improved infrastructure?
Data scientists can enhance their developer platform and infrastructure by utilizing the library to fetch data and process user inputs more effectively. By running pip install langchain
, they gain access to a robust set of tools that streamline the integration of external data sources, ensuring that applications are responsive and context-aware. This integration is key to building advanced AI models and prompt engineering solutions that can adapt to the evolving needs of users and businesses.
How does LangChain facilitate prompt engineering and management for AI models?
LC streamlines the development of end-to-end agents by providing robust tools for prompt engineering, which includes prompt management and optimization. With features like vector databases, LangChain enhances the output format of AI models, ensuring that the question answering capabilities are fine-tuned and highly responsive. By integrating these tools with other resources, LangChain empowers developers to build sophisticated AI-driven applications with ease.
How does LangChain support prompt optimization and agent involvement in an LLM framework?
As an open-source project it provides a comprehensive suite of building blocks for the development of sophisticated agents within an LLM framework. Written in both Python and JavaScript, it offers developers the tools necessary for prompt optimization, ensuring that specific documents and user queries are handled with precision. By leveraging these robust tools, LangChain empowers developers to create responsive and intelligent agents, enhancing the capabilities of LLMs in various applications.
What are the main value propositions of LangChain's available tools and third-party integrations?
LangChain's suite of available tools and third-party integrations offers a robust platform to connect AI models with external services, enhancing the capabilities of applications across various domains. With integrations like Google Search, our platform empowers the sales team and other users to leverage the power of AI in their workflows. The following modules within LangChain are designed to streamline the integration process and optimize the use of AI models, ensuring that our users can harness the full potential of their AI-driven solutions.
How does LangChain utilize multiple LLMs and vector embeddings to enhance artificial intelligence applications?
LangChain is important for the development of advanced artificial intelligence applications as it allows for the integration of multiple large language models (LLMs) and the use of vector embeddings. This integration enables LangChain to work with a rich variety of data sources, improving the system's understanding and response accuracy. By leveraging these capabilities, LangChain provides better documentation and support for developers, ensuring that AI applications are more robust, context-aware, and capable of handling complex user interactions.
How does LangChain enhance generative AI with common utilities and intermediate steps?
LangChain significantly enhances generative AI by providing full documentation and common utilities that streamline the generation step. This ensures that developers have detailed information and support for the intermediate steps involved in creating sophisticated AI applications. By being extremely open with its processes and resources, LangChain fosters an environment where generative AI can thrive and evolve.
How can LangChain's components and third-party integrations accelerate LLM application development?
LangChain's ecosystem is designed to accelerate the development of LLM applications by providing a suite of common utilities and other components that streamline the integration process. With these tools, developers can build applications more efficiently, bringing new features to production faster. The platform's extensive third-party integrations also ensure that applications can easily connect with a wide range of services, enhancing functionality and user experience.