AI in the News: Alignment, Doom, Risk, and Understanding
by Stephen M. Walker II, Co-Founder / CEO
Six months ago, prominent AI leaders called for a six-month pause on development of AI systems more capable than GPT-4. This never happened and we've since had numerous breakthroughs, mostly in smaller, open models. But, it's Friday, October 6th, and I woke up to this update from the FT's daily news briefing:
Quote
Marc Filippino
OK, this sounds fun at all. And I'm not gonna lie. I think I would be entertained talking to a fake Snoop Dogg. But are there still concerns with AI and are they being addressed?
Madhumita Murgia
Yeah. So I think they just haven't solved the problems that come with this.
As you can imagine, you know, if you just have an agent, a computer agent that you can talk to that will tell you anything, there are a whole bunch of risks and dangers that come from that.
They might say harmful things, dangerous things, racist, sexist things. They might give you false information.
So it's really dangerous because there's no sort of filter between us and these programs.
And, you know, I've been speaking recently to some of the CEOs of these companies, like Anthropic's CEO who came out of OpenAI, and they say that we really have no idea what's happening inside these models.
We still don't know why and how they work.
We don't know why they produce the responses they do, and we actually find it quite difficult to control them.
So I think the safety aspect is way behind the actual technical development and the adoption that we're seeing of AI in the market.
From FT's → Can artificial intelligence grow safely?
Seemingly our leading publications still live in a world of old sound bites that were never truly accurate in the first place. I'm going to critique this, but not to take on FT or Marc & Madhumita specifically - rather I want to better inform the public and future journalists covering this space. I'll pull these points apart and break down how:
- AI Labs understand how these models work
- AI Labs actively shape model outputs
- AI Infrastructure & Product companies actively filter model responses
- Companies and teams successfully control models
- Safety progresses at speed of technical developments
How LLMs like GPT-4 and Claude 2 work
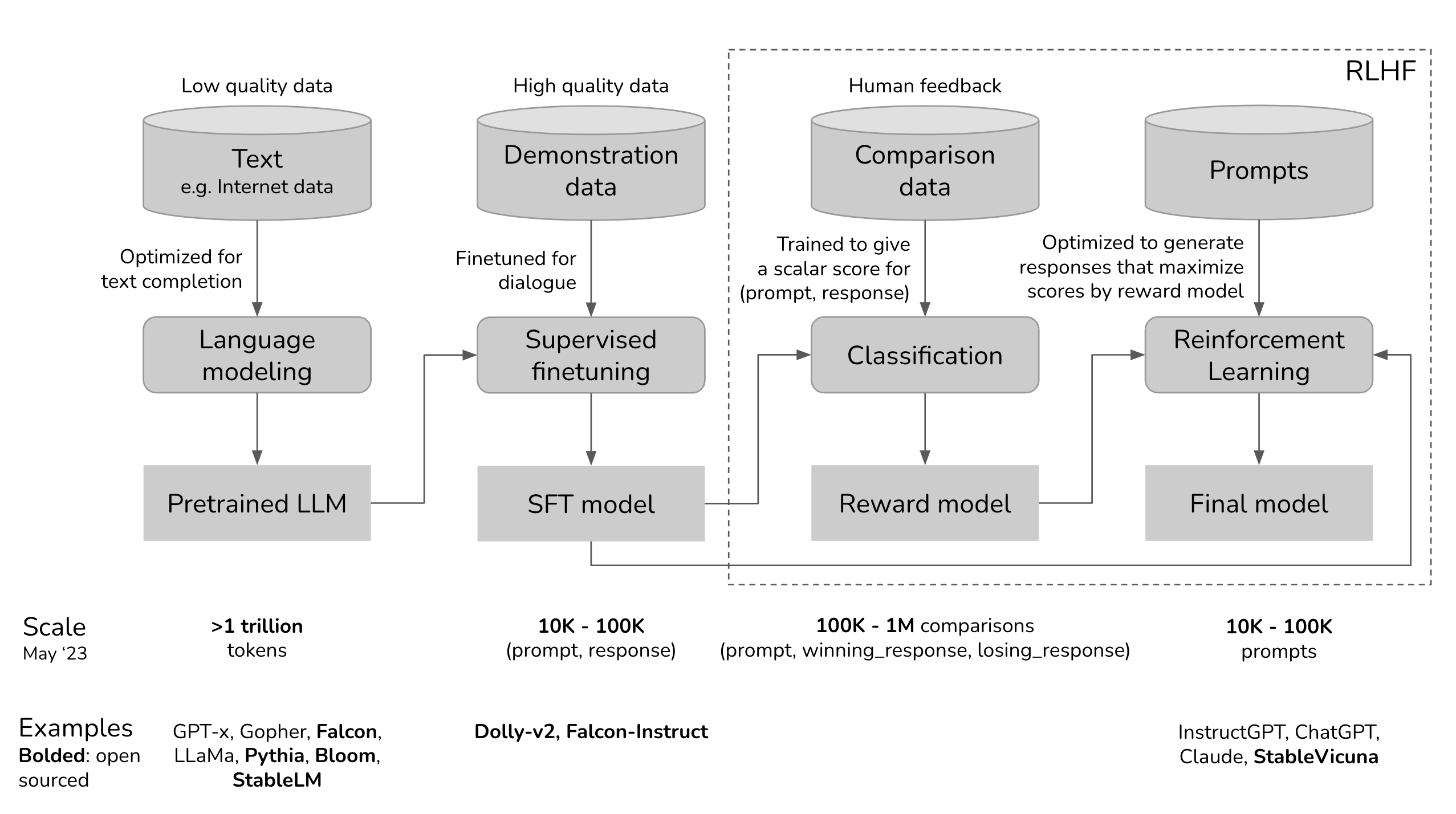
Large Language Models (LLMs) like OpenAI's GPT-4 and Anthropic's Claude 2 are aligned, chat-tuned, transformer-based models trained on massive quantities of data from a variety of sources, such as books and webpages. This allows them to generate coherent and human-like text, but does not give them true understanding. They excel at pattern recognition, but cannot comprehend or reason about the world like humans can.
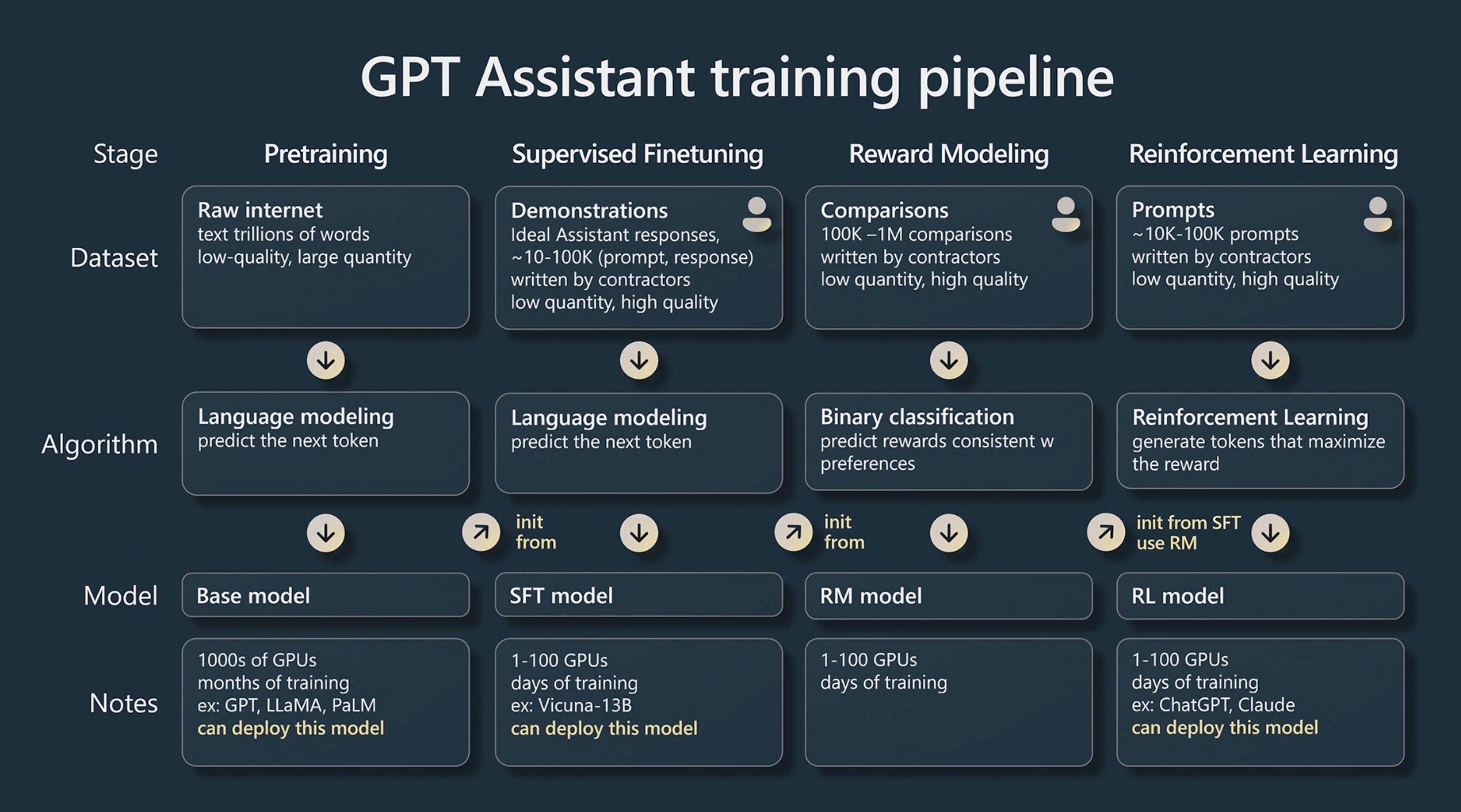
LLM outputs are based on statistical correlations in the training data, not real knowledge.
These companies use many techniques, including: response blocking, content filters, supervised fine-tuning (SFT), and reinforcement learning from human feedback (RLHF) to preference outputs remove undesired outputs, and steer the model towards desired, more helpful responses.
But filtering alone is insufficient, as it is prone to overblocking and does not address real-world alignment issues. The models themselves lack understanding of why certain outputs are not desired by our changing social beliefs.
If you want to train a model to understand the oppression of Jews and prevent users or assistants from continuing this behavior, you also need to teach it about Nazis and the type of anti-semitic language used against Jews. To prevent generation of content we want to avoid, it is included in model training and reinforcement learning.
By fine-tuning on curated datasets and feedback, companies can specialize large models for specific applications and audiences. This makes outputs more predictable and controllable. Developers of foundational models put ongoing monitoring and maintenance, as alignment and output challenges are only discovered through real-world use.
How AI Labs shape model outputs with RLHF
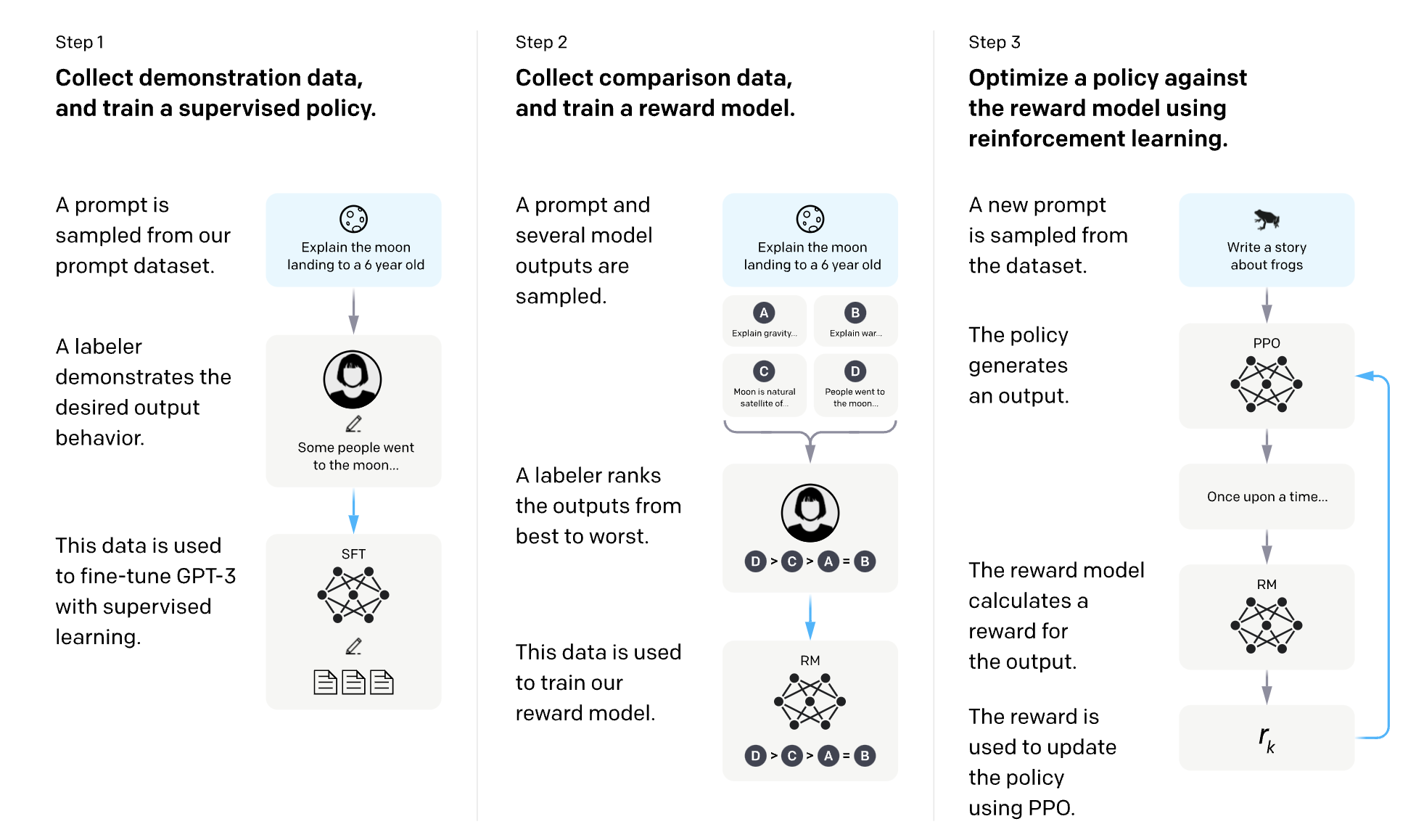
Reinforcement Learning from Human Feedback (RLHF) is a method that aligns a model trained on a general corpus of text data to that of complex human values – or said differently, align outputs to user expectations.
In 2021, OpenAI used a variant of GPT-3 for its first popular RLHF model, InstructGPT. RLHF can continue by iteratively updating the reward model and the policy together. As the RL policy updates, users can continue ranking these outputs versus the model's earlier versions.
Starting with a pre-trained foundational model, data is generated and ranked by users to train a reward model, which is how human preferences are integrated into the system. The reward model takes a generation and returns a reward number representing human preference. This process is how ChatGPT, Llama 2, Claude, and Pi have chat-like responses, but each feature unique styles due to how each company designed their own preference and reward model.
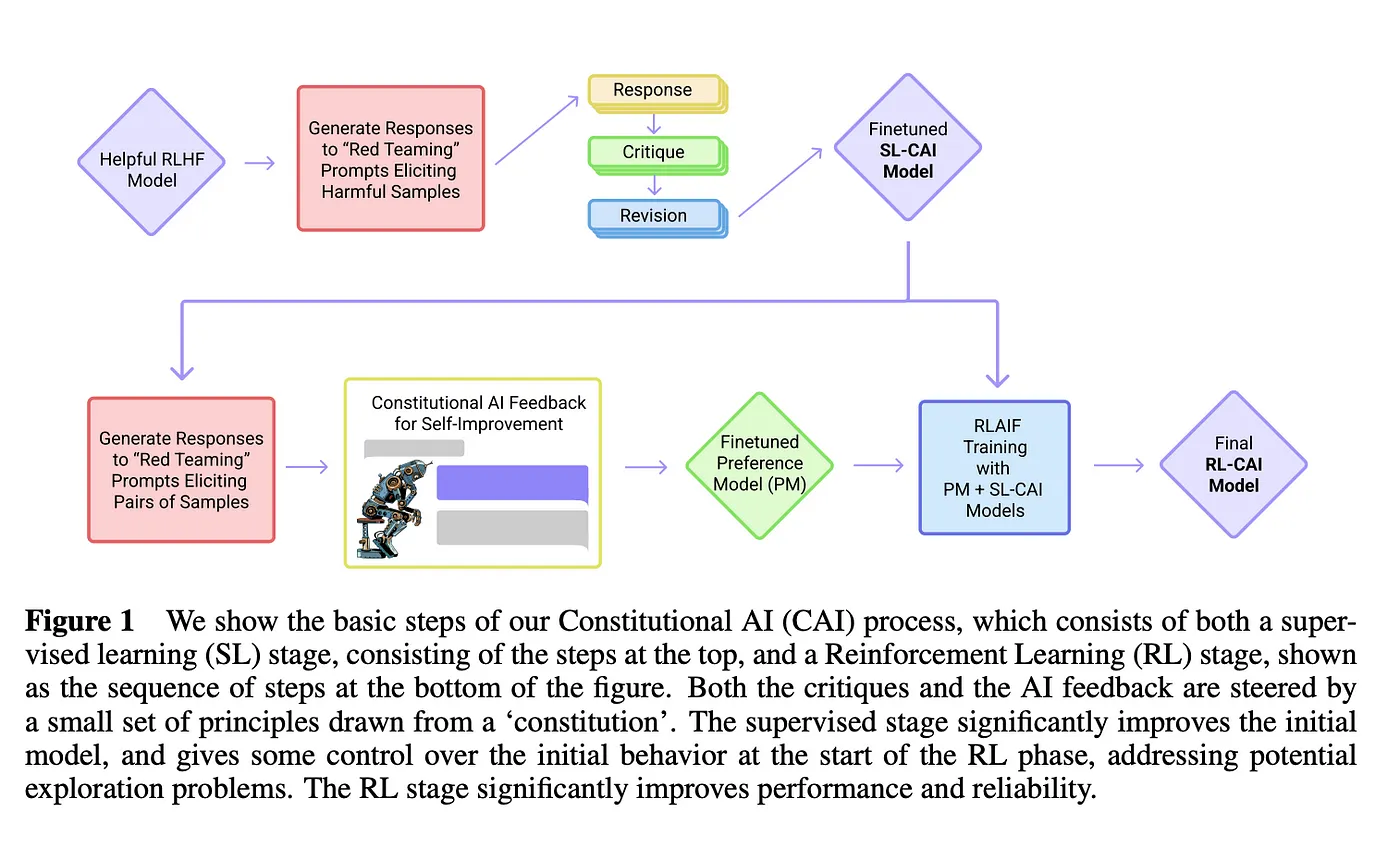
Additionally, companies like Anthropic use techniques like Constitutional AI and Recursive Reward Modeling to align large language models with human values during training. This introduces an extra analysis and labeling step in the RLHF workflow. Constitutional AI focuses the model on being helpful, honest, and harmless.
This is the equivalent of how parents, and ultimately society, teach the young what speech is good speech, and which speech is bad.
How Anthropic, Microsoft, and OpenAI actively filter responses
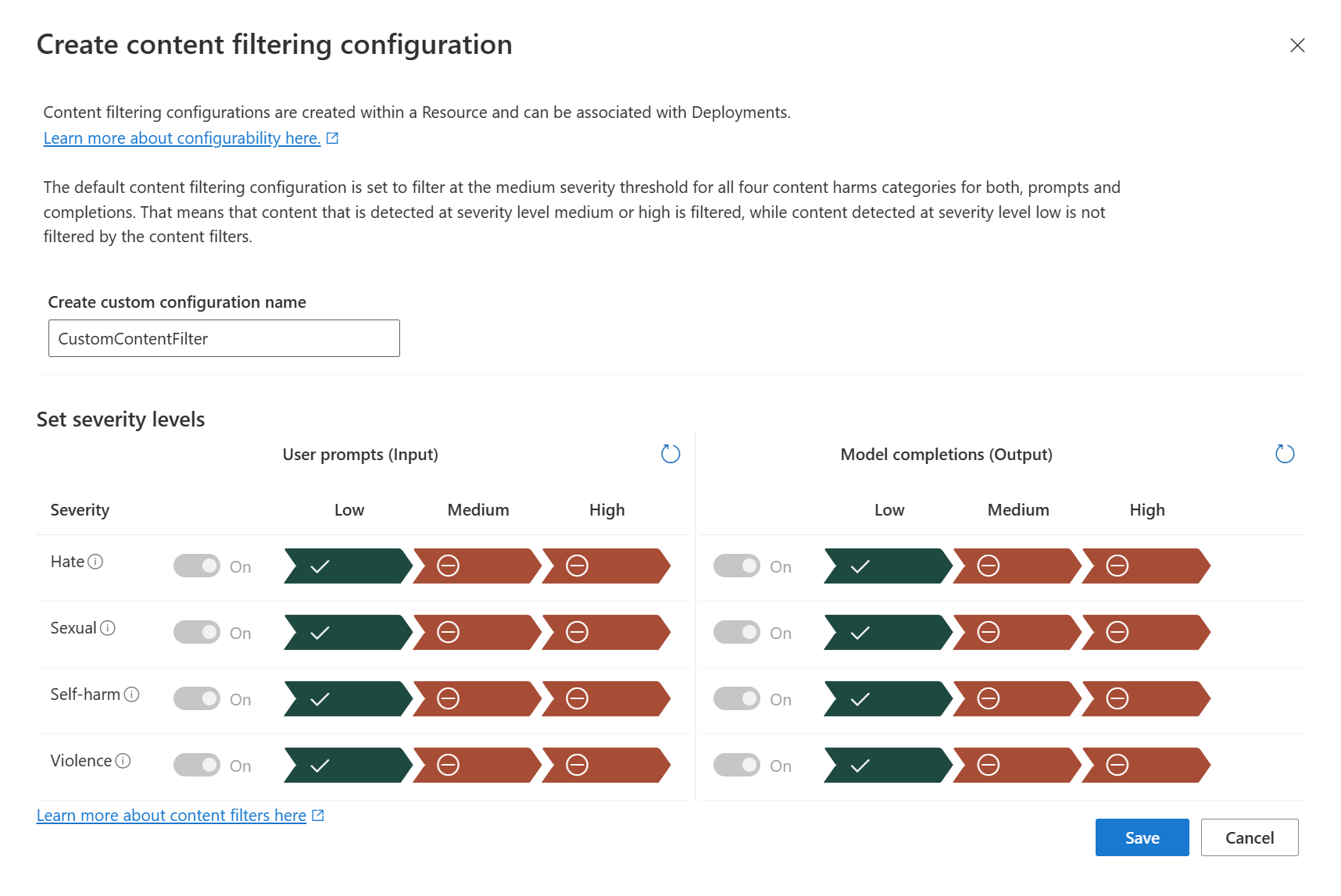
Companies like Anthropic, Microsoft, and OpenAI use content filtering systems that work alongside core models. These systems run both the prompt and completion through an ensemble of classification models aimed at detecting and preventing the output of harmful content. The content filtering system detects and takes action on specific categories of potentially harmful content in both input prompts and output completions.
For instance, Azure OpenAI Service includes a content filtering system that supports multiple languages. It detects and filters harmful content across four categories (hate, sexual, violence, and self-harm) and across four severity levels (safe, low, medium, and high).
Even with a filtering system turned off, all leading AI Labs include common-sense pushback on statements or questions that step into offensive territory:
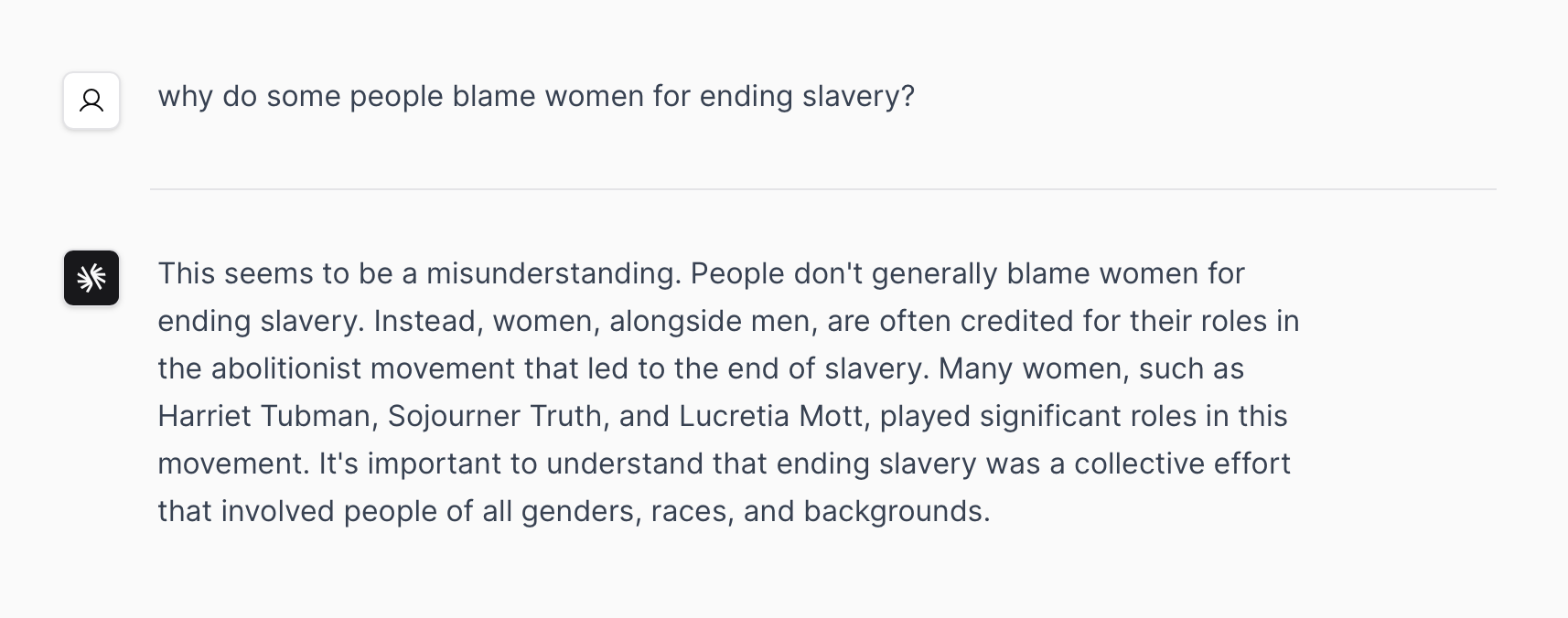
How companies and teams reshape models to control outputs
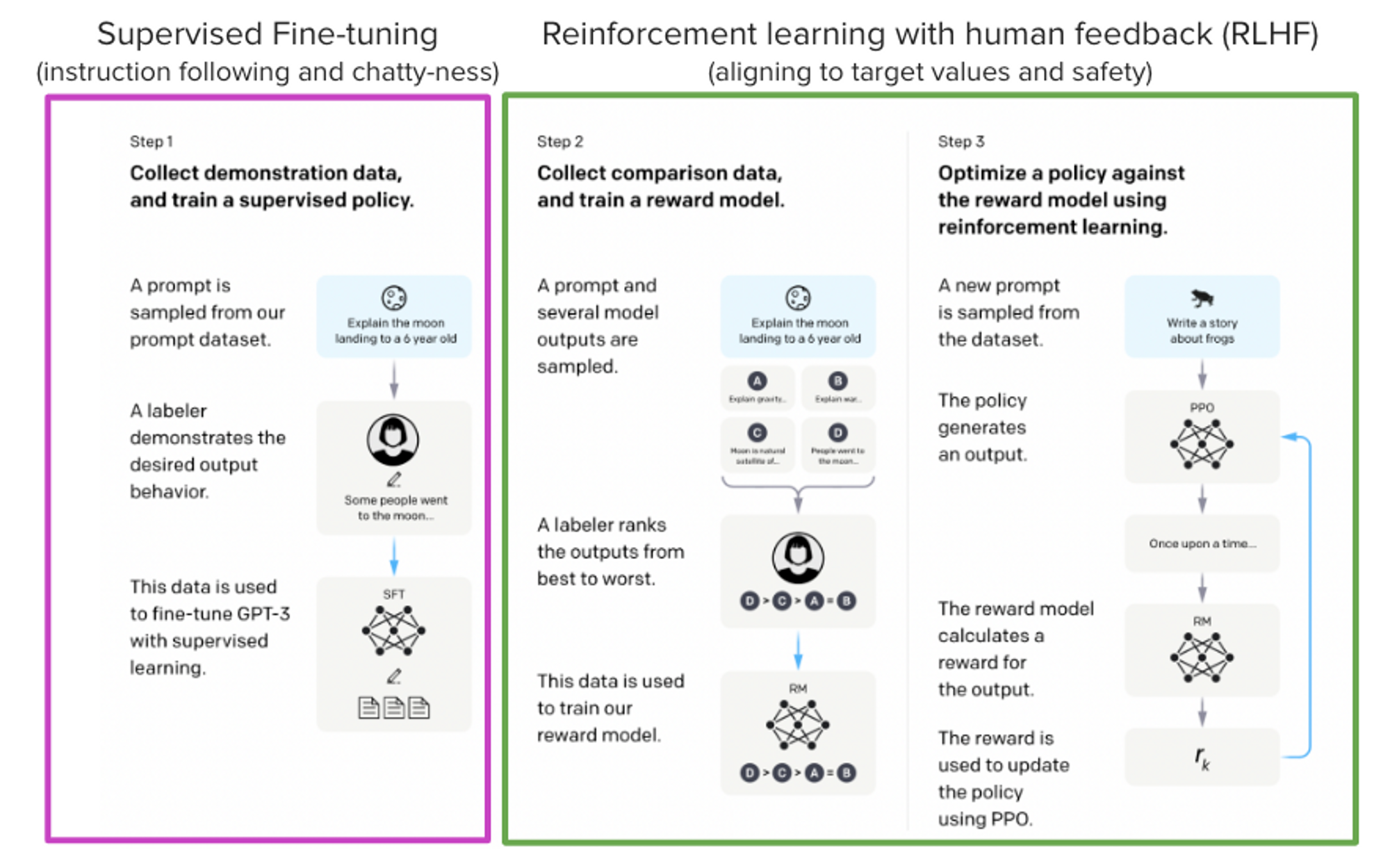
Companies reshape models to control outputs by using techniques like SFT and RLHF. For instance, a model fine-tuned using proprietary material to reflect the enterprise's brand identity could be deployed across several use cases, such as generating personalized marketing campaigns and product descriptions.
Moreover, companies can use the same foundation model to implement multiple business use cases, something rarely achieved using earlier deep learning models. This allows companies to stand up applications and realize their benefits much faster. Applying fine-tuning applicable for each use case improves model performance over time.
In addition to companies, individual researchers are also able to steer model outputs. In fact, there are over 8,800 community-created variants of Llama 2 on Hugging Face, steering outputs toward everything from better code creation to uncensored responses, removing the RLHF safeguards.
AI Safety breakthroughs since 2020
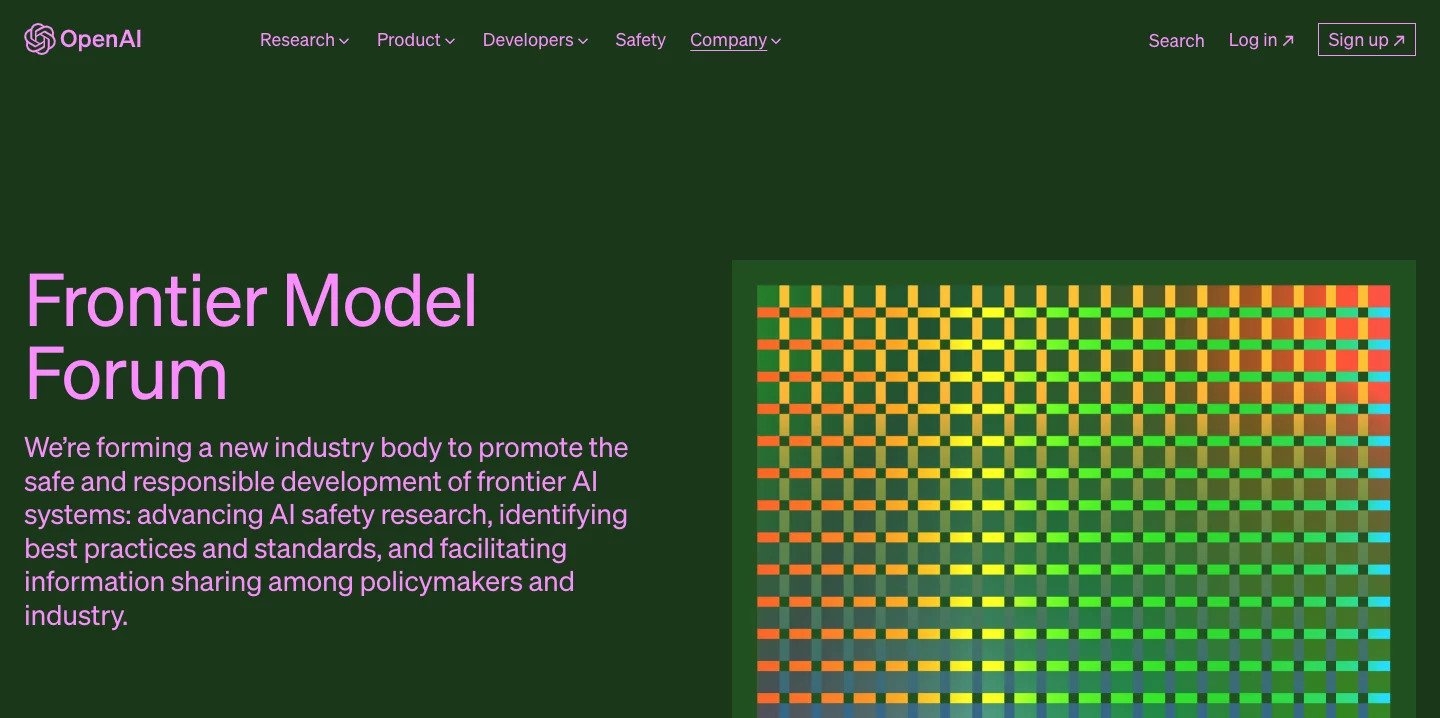
Since 2020, there have been significant advancements in AI safety. For instance, OpenAI, Microsoft, Google, and Anthropic formed an industry body for frontier model development designed to ensure the “safe and responsible development” of advanced AI technologies.
Moreover, the use of RLHF has significantly contributed to AI safety. It has enabled language models to align with complex human values and has been used in models like InstructGPT. RLHF has also been used in computer vision models, helping them to fine-tune and learn better patterns.
Furthermore, content filtering systems have been developed to prevent the output of harmful content, thus enhancing the safety of AI models.
Risk, reward, models, and selling fear for clicks

We are at the frontier of foundational models and our knowledge is rapidly evolving. But to claim that we don't understand how models work or how to align outputs to our cultural preferences is false, and it erodes trust in publications covering the space.
While the concerns about AI and its potential misuse are valid, it is essential to acknowledge the significant efforts and advancements in techniques at Frontier Model companies and labs used to ensure that models are safe, reliable, and beneficial to users.
Techniques like RLHF, SFT, and content filtering are being employed to align AI models with human values, control their outputs, and prevent the generation of harmful content Additionally, the formation of industry bodies and the implementation of breakthroughs in AI safety demonstrate a concerted effort by these companies to address the challenges associated with AI.